class: center, middle, inverse, title-slide # Statistical Inference and Data Exploration for Archaeologists ## A Guide to using R for Beginners ### Ben Marwick, June 2020 --- class: center, left .pull-left[ <img class="mask" /> ] .pull-right[ # Find me at... .left[ [<svg style="height:0.8em;top:.04em;position:relative;" viewBox="0 0 512 512"><path d="M459.37 151.716c.325 4.548.325 9.097.325 13.645 0 138.72-105.583 298.558-298.558 298.558-59.452 0-114.68-17.219-161.137-47.106 8.447.974 16.568 1.299 25.34 1.299 49.055 0 94.213-16.568 130.274-44.832-46.132-.975-84.792-31.188-98.112-72.772 6.498.974 12.995 1.624 19.818 1.624 9.421 0 18.843-1.3 27.614-3.573-48.081-9.747-84.143-51.98-84.143-102.985v-1.299c13.969 7.797 30.214 12.67 47.431 13.319-28.264-18.843-46.781-51.005-46.781-87.391 0-19.492 5.197-37.36 14.294-52.954 51.655 63.675 129.3 105.258 216.365 109.807-1.624-7.797-2.599-15.918-2.599-24.04 0-57.828 46.782-104.934 104.934-104.934 30.213 0 57.502 12.67 76.67 33.137 23.715-4.548 46.456-13.32 66.599-25.34-7.798 24.366-24.366 44.833-46.132 57.827 21.117-2.273 41.584-8.122 60.426-16.243-14.292 20.791-32.161 39.308-52.628 54.253z"/></svg> @benmarwick](http://twitter.com/benmarwick) [<svg style="height:0.8em;top:.04em;position:relative;" viewBox="0 0 496 512"><path d="M165.9 397.4c0 2-2.3 3.6-5.2 3.6-3.3.3-5.6-1.3-5.6-3.6 0-2 2.3-3.6 5.2-3.6 3-.3 5.6 1.3 5.6 3.6zm-31.1-4.5c-.7 2 1.3 4.3 4.3 4.9 2.6 1 5.6 0 6.2-2s-1.3-4.3-4.3-5.2c-2.6-.7-5.5.3-6.2 2.3zm44.2-1.7c-2.9.7-4.9 2.6-4.6 4.9.3 2 2.9 3.3 5.9 2.6 2.9-.7 4.9-2.6 4.6-4.6-.3-1.9-3-3.2-5.9-2.9zM244.8 8C106.1 8 0 113.3 0 252c0 110.9 69.8 205.8 169.5 239.2 12.8 2.3 17.3-5.6 17.3-12.1 0-6.2-.3-40.4-.3-61.4 0 0-70 15-84.7-29.8 0 0-11.4-29.1-27.8-36.6 0 0-22.9-15.7 1.6-15.4 0 0 24.9 2 38.6 25.8 21.9 38.6 58.6 27.5 72.9 20.9 2.3-16 8.8-27.1 16-33.7-55.9-6.2-112.3-14.3-112.3-110.5 0-27.5 7.6-41.3 23.6-58.9-2.6-6.5-11.1-33.3 2.6-67.9 20.9-6.5 69 27 69 27 20-5.6 41.5-8.5 62.8-8.5s42.8 2.9 62.8 8.5c0 0 48.1-33.6 69-27 13.7 34.7 5.2 61.4 2.6 67.9 16 17.7 25.8 31.5 25.8 58.9 0 96.5-58.9 104.2-114.8 110.5 9.2 7.9 17 22.9 17 46.4 0 33.7-.3 75.4-.3 83.6 0 6.5 4.6 14.4 17.3 12.1C428.2 457.8 496 362.9 496 252 496 113.3 383.5 8 244.8 8zM97.2 352.9c-1.3 1-1 3.3.7 5.2 1.6 1.6 3.9 2.3 5.2 1 1.3-1 1-3.3-.7-5.2-1.6-1.6-3.9-2.3-5.2-1zm-10.8-8.1c-.7 1.3.3 2.9 2.3 3.9 1.6 1 3.6.7 4.3-.7.7-1.3-.3-2.9-2.3-3.9-2-.6-3.6-.3-4.3.7zm32.4 35.6c-1.6 1.3-1 4.3 1.3 6.2 2.3 2.3 5.2 2.6 6.5 1 1.3-1.3.7-4.3-1.3-6.2-2.2-2.3-5.2-2.6-6.5-1zm-11.4-14.7c-1.6 1-1.6 3.6 0 5.9 1.6 2.3 4.3 3.3 5.6 2.3 1.6-1.3 1.6-3.9 0-6.2-1.4-2.3-4-3.3-5.6-2z"/></svg> @benmarwick](http://github.com/benmarwick) [<svg style="height:0.8em;top:.04em;position:relative;" viewBox="0 0 512 512"><path d="M326.612 185.391c59.747 59.809 58.927 155.698.36 214.59-.11.12-.24.25-.36.37l-67.2 67.2c-59.27 59.27-155.699 59.262-214.96 0-59.27-59.26-59.27-155.7 0-214.96l37.106-37.106c9.84-9.84 26.786-3.3 27.294 10.606.648 17.722 3.826 35.527 9.69 52.721 1.986 5.822.567 12.262-3.783 16.612l-13.087 13.087c-28.026 28.026-28.905 73.66-1.155 101.96 28.024 28.579 74.086 28.749 102.325.51l67.2-67.19c28.191-28.191 28.073-73.757 0-101.83-3.701-3.694-7.429-6.564-10.341-8.569a16.037 16.037 0 0 1-6.947-12.606c-.396-10.567 3.348-21.456 11.698-29.806l21.054-21.055c5.521-5.521 14.182-6.199 20.584-1.731a152.482 152.482 0 0 1 20.522 17.197zM467.547 44.449c-59.261-59.262-155.69-59.27-214.96 0l-67.2 67.2c-.12.12-.25.25-.36.37-58.566 58.892-59.387 154.781.36 214.59a152.454 152.454 0 0 0 20.521 17.196c6.402 4.468 15.064 3.789 20.584-1.731l21.054-21.055c8.35-8.35 12.094-19.239 11.698-29.806a16.037 16.037 0 0 0-6.947-12.606c-2.912-2.005-6.64-4.875-10.341-8.569-28.073-28.073-28.191-73.639 0-101.83l67.2-67.19c28.239-28.239 74.3-28.069 102.325.51 27.75 28.3 26.872 73.934-1.155 101.96l-13.087 13.087c-4.35 4.35-5.769 10.79-3.783 16.612 5.864 17.194 9.042 34.999 9.69 52.721.509 13.906 17.454 20.446 27.294 10.606l37.106-37.106c59.271-59.259 59.271-155.699.001-214.959z"/></svg> faculty.washington.edu/bmarwick](http://faculty.washington.edu/bmarwick) [<svg style="height:0.8em;top:.04em;position:relative;" viewBox="0 0 512 512"><path d="M476 3.2L12.5 270.6c-18.1 10.4-15.8 35.6 2.2 43.2L121 358.4l287.3-253.2c5.5-4.9 13.3 2.6 8.6 8.3L176 407v80.5c0 23.6 28.5 32.9 42.5 15.8L282 426l124.6 52.2c14.2 6 30.4-2.9 33-18.2l72-432C515 7.8 493.3-6.8 476 3.2z"/></svg> bmarwick@uw.edu](mailto:bmarwick@uw.edu) ] ] --- # Welcome - Statistical inference is the process of making conclusions from a sample of data -- - One kind of statistical inference is hypothesis testing, where we use a sample of data to determine the strength of evidence for or against our theory -- - Data exploration is searching for patterns, groups and characteristics in our sample -- - We are going to do statistical inference and data exploration using the tidyverse (to catch up, see [tidyverse for archaeologists](https://github.com/benmarwick/tidyverse-for-archaeology)) -- - When we write R code to analyse archaeological data, our analysis is **transparent and reproducible**. These are two vital characteristics of good scientific work. -- - We should **share our code and data** with our research publications for others to study and learn --- # What are we going to do today? I will demonstrate and you will practice... -- - Hypothesis testing: chi-square, t-test, ANOVA -- - Exploration: Principal Components Analysis -- - Exploration: k-means clustering --- class: center, middle # Let's import some archaeological data 💽 --- class: left, middle background-image: url(figures/data-source-paper.png) background-size: contain --- class: split-40 count: false .left-code-import-auto[ ```r *library(rio) ``` ] .right-output-import-auto[ ] --- class: split-40 count: false .left-code-import-auto[ ```r library(rio) # read in the data *j_data <- import("https://bit.ly/j_data_xlsx", setclass = "tbl_df") ``` ] .right-output-import-auto[ ] --- class: split-40 count: false .left-code-import-auto[ ```r library(rio) # read in the data j_data <- import("https://bit.ly/j_data_xlsx", setclass = "tbl_df") # take a look *j_data ``` ] .right-output-import-auto[ ``` # A tibble: 9,752 x 48 Site Square Spit Group Artno Material Colour Weight Length Artclas Cortex Cortype Initiat Breaks Noseg <chr> <chr> <dbl> <dbl> <dbl> <chr> <chr> <dbl> <dbl> <chr> <dbl> <chr> <chr> <chr> <dbl> 1 J B 1 1 1 Chert Brown 1.83 22.0 Flake 0 <NA> Hertz <NA> NA 2 J B 1 1 2 Chert Yellow 0.61 14.6 RetF 0 <NA> Hertz <NA> 3 3 J B 1 1 3 Chert Dk Gr… 3.81 29.6 Core 0 <NA> <NA> <NA> NA 4 J B 1 1 4 Chert Grey 0.13 5.83 RetFrag 0 <NA> <NA> Both 1 5 J B 1 1 5 Chert Grey 0.05 7.07 Flake 0 <NA> Hertz <NA> NA 6 J B 1 1 6 Chert Lt Br… 0.580 11.1 Core NA <NA> <NA> <NA> NA 7 J B 1 1 7 Chert Dk Br… 0.03 5.72 Flake 0 <NA> Hertz <NA> NA 8 J B 1 1 8 Chert Cream 0.02 8.37 FFrag 0 <NA> <NA> <NA> NA 9 J B 1 1 9 Chert Grey 0.21 10.7 Flake-b 0 <NA> Hertz Long NA 10 J B 1 1 10 Chert Grey 0.25 14.3 Flake 0 <NA> Hertz <NA> NA # … with 9,742 more rows, and 33 more variables: Platwid <dbl>, Plat <chr>, Focal <lgl>, Overhang <chr>, # NoDS <dbl>, NoPS <dbl>, Rtch <chr>, Plat_2 <chr>, Focal_2 <lgl>, Overhang_2 <chr>, NoDS_2 <dbl>, # NoPS_2 <dbl>, Rtch_2 <chr>, RetOri <chr>, Retype <chr>, Retloc <chr>, Retlen <dbl>, Retdep <dbl>, # Portion <chr>, Heat <dbl>, EdgeDam <dbl>, Weathering <dbl>, Corerot <dbl>, RetScNo <dbl>, Term <chr>, # Janus <lgl>, Recycled <lgl>, Redirect <lgl>, Width <dbl>, Thick <dbl>, Platthic <dbl>, area <dbl>, # elongation <dbl> ``` ] <style> .left-code-import-auto { color: #777; width: 38%; height: 92%; float: left; font-size: 80% } .right-output-import-auto { width: 60%; float: right; padding-left: 1%; } </style> --- class: left, middle background-image: url(https://images.unsplash.com/photo-1515490480959-ce9152f7ea2b?ixlib=rb-1.2.1&ixid=eyJhcHBfaWQiOjEyMDd9&auto=format&fit=crop&w=2850&q=80) background-size: cover --- # Your turn: import the data ```r library(rio) # read in the data j_data <- import("https://bit.ly/j_data_xlsx", setclass = "tbl_df") # take a look j_data ```
02
:
00
--- class: center, middle # Let's do some hypothesis testing 🧪 We will use the `infer` package and the [Modern Dive](https://moderndive.com/) book by Chester Ismay and Albert Y. Kim --- # This really is only one test [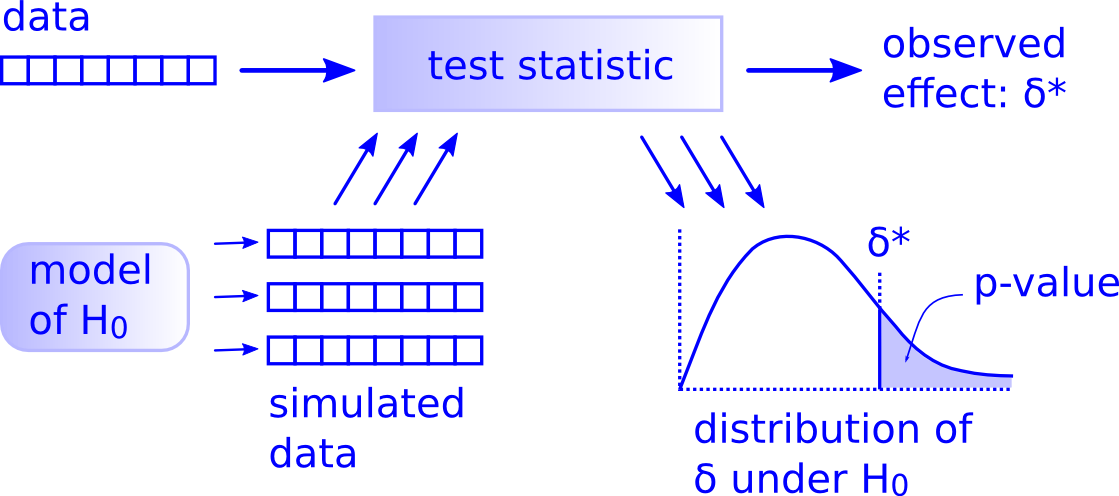](http://allendowney.blogspot.com/2016/06/there-is-still-only-one-test.html) --- # Here are the basic steps 👣 [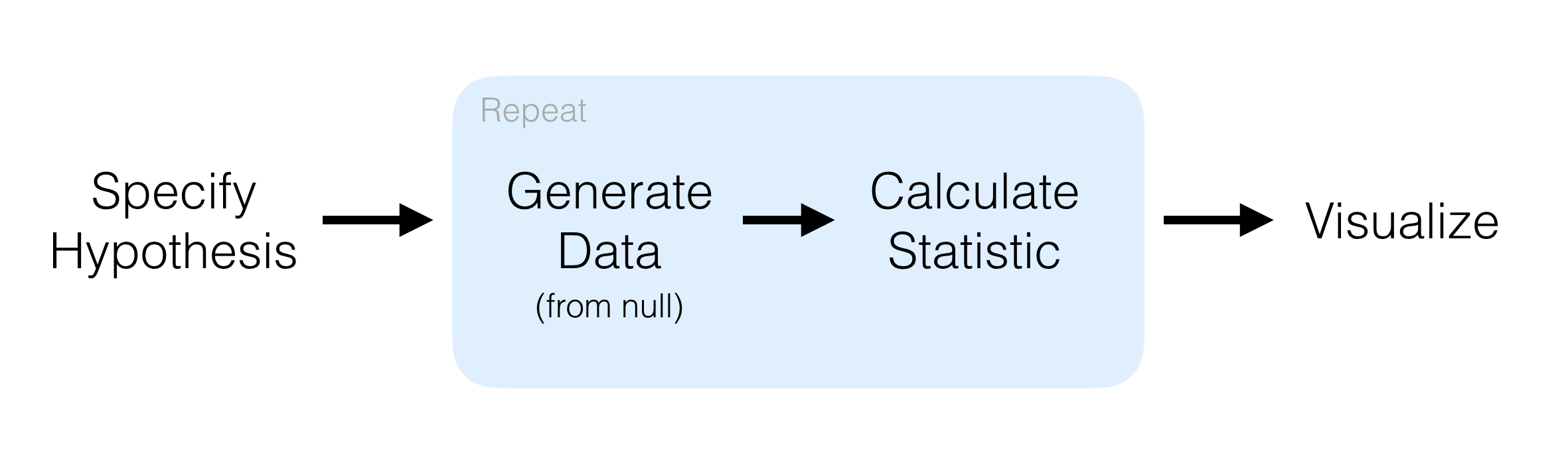](https://infer.netlify.app/) --- class: left, bottom background-image: url(figures/infer.073.jpeg) background-size: contain Start with our data --- class: left, bottom background-image: url(figures/infer.074.jpeg) background-size: contain Specify the variables in our data frame that we want to investigate --- class: left, bottom background-image: url(figures/infer.075.jpeg) background-size: contain --- class: left, bottom background-image: url(figures/infer.076.jpeg) background-size: contain Declare the null hypothesis, the observed effect was simply due to random chance --- class: left, bottom background-image: url(figures/infer.077.jpeg) background-size: contain --- class: left, bottom background-image: url(figures/infer.078.jpeg) background-size: contain Generate many datasets according to the null hypothesis --- class: left, bottom background-image: url(figures/infer.079.jpeg) background-size: contain --- class: left, bottom background-image: url(figures/infer.081.jpeg) background-size: contain Calculate the distribution of the statistic from the generated datasets to create the null distribution. --- class: left, bottom background-image: url(figures/infer.082.jpeg) background-size: contain Visualize the statistic relative to the null distribution of our generated data --- # Let's do a chi-square test for the independence of raw material type and artefact type of our stone artefacts. -- # Is the association between artefact type and raw material type random or independent? --- class: split-40 count: false Prepare our data for a chi-square test: Is flake type independant from raw material type? .left-code-test-chi-square-1-auto[ ```r *library(tidyverse) ``` ] .right-output-test-chi-square-1-auto[ ] --- class: split-40 count: false Prepare our data for a chi-square test: Is flake type independant from raw material type? .left-code-test-chi-square-1-auto[ ```r library(tidyverse) # filter the data to # focus on our question *j_data ``` ] .right-output-test-chi-square-1-auto[ ``` # A tibble: 9,752 x 48 Site Square Spit Group Artno Material Colour Weight Length Artclas Cortex Cortype Initiat Breaks Noseg <chr> <chr> <dbl> <dbl> <dbl> <chr> <chr> <dbl> <dbl> <chr> <dbl> <chr> <chr> <chr> <dbl> 1 J B 1 1 1 Chert Brown 1.83 22.0 Flake 0 <NA> Hertz <NA> NA 2 J B 1 1 2 Chert Yellow 0.61 14.6 RetF 0 <NA> Hertz <NA> 3 3 J B 1 1 3 Chert Dk Gr… 3.81 29.6 Core 0 <NA> <NA> <NA> NA 4 J B 1 1 4 Chert Grey 0.13 5.83 RetFrag 0 <NA> <NA> Both 1 5 J B 1 1 5 Chert Grey 0.05 7.07 Flake 0 <NA> Hertz <NA> NA 6 J B 1 1 6 Chert Lt Br… 0.580 11.1 Core NA <NA> <NA> <NA> NA 7 J B 1 1 7 Chert Dk Br… 0.03 5.72 Flake 0 <NA> Hertz <NA> NA 8 J B 1 1 8 Chert Cream 0.02 8.37 FFrag 0 <NA> <NA> <NA> NA 9 J B 1 1 9 Chert Grey 0.21 10.7 Flake-b 0 <NA> Hertz Long NA 10 J B 1 1 10 Chert Grey 0.25 14.3 Flake 0 <NA> Hertz <NA> NA # … with 9,742 more rows, and 33 more variables: Platwid <dbl>, Plat <chr>, Focal <lgl>, Overhang <chr>, # NoDS <dbl>, NoPS <dbl>, Rtch <chr>, Plat_2 <chr>, Focal_2 <lgl>, Overhang_2 <chr>, NoDS_2 <dbl>, # NoPS_2 <dbl>, Rtch_2 <chr>, RetOri <chr>, Retype <chr>, Retloc <chr>, Retlen <dbl>, Retdep <dbl>, # Portion <chr>, Heat <dbl>, EdgeDam <dbl>, Weathering <dbl>, Corerot <dbl>, RetScNo <dbl>, Term <chr>, # Janus <lgl>, Recycled <lgl>, Redirect <lgl>, Width <dbl>, Thick <dbl>, Platthic <dbl>, area <dbl>, # elongation <dbl> ``` ] --- class: split-40 count: false Prepare our data for a chi-square test: Is flake type independant from raw material type? .left-code-test-chi-square-1-auto[ ```r library(tidyverse) # filter the data to # focus on our question j_data %>% * filter(Material %in% c("Volcanic", "Quartzite")) ``` ] .right-output-test-chi-square-1-auto[ ``` # A tibble: 475 x 48 Site Square Spit Group Artno Material Colour Weight Length Artclas Cortex Cortype Initiat Breaks Noseg <chr> <chr> <dbl> <dbl> <dbl> <chr> <chr> <dbl> <dbl> <chr> <dbl> <chr> <chr> <chr> <dbl> 1 J B 1 1 15 Volcanic Grey 1.07 6.36 Flake-b 20 Angul Hertz Long NA 2 J B 1 1 33 Volcanic Brown 0.23 8.5 Flake 0 <NA> Bending <NA> NA 3 J B 1 1 38 Volcanic Dk Gr… 0.06 4.24 Flake 60 Angul Hertz <NA> NA 4 J B 1 1 59 Volcanic Dk Gr… 0.43 12.9 Shatt 0 <NA> <NA> <NA> NA 5 J B 1 1 70 Volcanic Dk Br… 0.31 7.43 Hshat NA <NA> <NA> <NA> NA 6 J B 1 1 71 Volcanic Dk Br… 0.26 6.4 Hshat NA <NA> <NA> <NA> NA 7 J B 1 1 72 Volcanic Dk Br… 0.06 7.3 Hshat NA <NA> <NA> <NA> NA 8 J B 1 1 73 Volcanic Dk Br… 0.16 7.84 Hshat NA <NA> <NA> <NA> NA 9 J B 1 1 78 Volcanic Lt Br… 0.02 6.47 Hshat NA <NA> <NA> <NA> NA 10 J B 2 1 88 Volcanic Grey 0.38 13.3 Flake 40 Angul Hertz <NA> NA # … with 465 more rows, and 33 more variables: Platwid <dbl>, Plat <chr>, Focal <lgl>, Overhang <chr>, # NoDS <dbl>, NoPS <dbl>, Rtch <chr>, Plat_2 <chr>, Focal_2 <lgl>, Overhang_2 <chr>, NoDS_2 <dbl>, # NoPS_2 <dbl>, Rtch_2 <chr>, RetOri <chr>, Retype <chr>, Retloc <chr>, Retlen <dbl>, Retdep <dbl>, # Portion <chr>, Heat <dbl>, EdgeDam <dbl>, Weathering <dbl>, Corerot <dbl>, RetScNo <dbl>, Term <chr>, # Janus <lgl>, Recycled <lgl>, Redirect <lgl>, Width <dbl>, Thick <dbl>, Platthic <dbl>, area <dbl>, # elongation <dbl> ``` ] --- class: split-40 count: false Prepare our data for a chi-square test: Is flake type independant from raw material type? .left-code-test-chi-square-1-auto[ ```r library(tidyverse) # filter the data to # focus on our question j_data %>% filter(Material %in% c("Volcanic", "Quartzite")) %>% * filter(Artclas %in% c("Flake", "Hshat", "FFrag")) ``` ] .right-output-test-chi-square-1-auto[ ``` # A tibble: 297 x 48 Site Square Spit Group Artno Material Colour Weight Length Artclas Cortex Cortype Initiat Breaks Noseg <chr> <chr> <dbl> <dbl> <dbl> <chr> <chr> <dbl> <dbl> <chr> <dbl> <chr> <chr> <chr> <dbl> 1 J B 1 1 33 Volcanic Brown 0.23 8.5 Flake 0 <NA> Bending <NA> NA 2 J B 1 1 38 Volcanic Dk Gr… 0.06 4.24 Flake 60 Angul Hertz <NA> NA 3 J B 1 1 70 Volcanic Dk Br… 0.31 7.43 Hshat NA <NA> <NA> <NA> NA 4 J B 1 1 71 Volcanic Dk Br… 0.26 6.4 Hshat NA <NA> <NA> <NA> NA 5 J B 1 1 72 Volcanic Dk Br… 0.06 7.3 Hshat NA <NA> <NA> <NA> NA 6 J B 1 1 73 Volcanic Dk Br… 0.16 7.84 Hshat NA <NA> <NA> <NA> NA 7 J B 1 1 78 Volcanic Lt Br… 0.02 6.47 Hshat NA <NA> <NA> <NA> NA 8 J B 2 1 88 Volcanic Grey 0.38 13.3 Flake 40 Angul Hertz <NA> NA 9 J B 3 1 130 Volcanic Grey 17.8 38.4 Flake 0 <NA> Hertz <NA> NA 10 J B 3 1 131 Volcanic Grey 32.1 57.4 Flake 40 Round <NA> <NA> NA # … with 287 more rows, and 33 more variables: Platwid <dbl>, Plat <chr>, Focal <lgl>, Overhang <chr>, # NoDS <dbl>, NoPS <dbl>, Rtch <chr>, Plat_2 <chr>, Focal_2 <lgl>, Overhang_2 <chr>, NoDS_2 <dbl>, # NoPS_2 <dbl>, Rtch_2 <chr>, RetOri <chr>, Retype <chr>, Retloc <chr>, Retlen <dbl>, Retdep <dbl>, # Portion <chr>, Heat <dbl>, EdgeDam <dbl>, Weathering <dbl>, Corerot <dbl>, RetScNo <dbl>, Term <chr>, # Janus <lgl>, Recycled <lgl>, Redirect <lgl>, Width <dbl>, Thick <dbl>, Platthic <dbl>, area <dbl>, # elongation <dbl> ``` ] --- class: split-40 count: false Prepare our data for a chi-square test: Is flake type independant from raw material type? .left-code-test-chi-square-1-auto[ ```r library(tidyverse) # filter the data to # focus on our question j_data %>% filter(Material %in% c("Volcanic", "Quartzite")) %>% filter(Artclas %in% c("Flake", "Hshat", "FFrag")) # plot the data *ggplot(j_data1) ``` ] .right-output-test-chi-square-1-auto[ ``` # A tibble: 297 x 48 Site Square Spit Group Artno Material Colour Weight Length Artclas Cortex Cortype Initiat Breaks Noseg <chr> <chr> <dbl> <dbl> <dbl> <chr> <chr> <dbl> <dbl> <chr> <dbl> <chr> <chr> <chr> <dbl> 1 J B 1 1 33 Volcanic Brown 0.23 8.5 Flake 0 <NA> Bending <NA> NA 2 J B 1 1 38 Volcanic Dk Gr… 0.06 4.24 Flake 60 Angul Hertz <NA> NA 3 J B 1 1 70 Volcanic Dk Br… 0.31 7.43 Hshat NA <NA> <NA> <NA> NA 4 J B 1 1 71 Volcanic Dk Br… 0.26 6.4 Hshat NA <NA> <NA> <NA> NA 5 J B 1 1 72 Volcanic Dk Br… 0.06 7.3 Hshat NA <NA> <NA> <NA> NA 6 J B 1 1 73 Volcanic Dk Br… 0.16 7.84 Hshat NA <NA> <NA> <NA> NA 7 J B 1 1 78 Volcanic Lt Br… 0.02 6.47 Hshat NA <NA> <NA> <NA> NA 8 J B 2 1 88 Volcanic Grey 0.38 13.3 Flake 40 Angul Hertz <NA> NA 9 J B 3 1 130 Volcanic Grey 17.8 38.4 Flake 0 <NA> Hertz <NA> NA 10 J B 3 1 131 Volcanic Grey 32.1 57.4 Flake 40 Round <NA> <NA> NA # … with 287 more rows, and 33 more variables: Platwid <dbl>, Plat <chr>, Focal <lgl>, Overhang <chr>, # NoDS <dbl>, NoPS <dbl>, Rtch <chr>, Plat_2 <chr>, Focal_2 <lgl>, Overhang_2 <chr>, NoDS_2 <dbl>, # NoPS_2 <dbl>, Rtch_2 <chr>, RetOri <chr>, Retype <chr>, Retloc <chr>, Retlen <dbl>, Retdep <dbl>, # Portion <chr>, Heat <dbl>, EdgeDam <dbl>, Weathering <dbl>, Corerot <dbl>, RetScNo <dbl>, Term <chr>, # Janus <lgl>, Recycled <lgl>, Redirect <lgl>, Width <dbl>, Thick <dbl>, Platthic <dbl>, area <dbl>, # elongation <dbl> ``` <img src="stat-inference-and-exploration-for-archaeologists_files/figure-html/test-chi-square-1_auto_5_output-1.png" width="432" /> ] --- class: split-40 count: false Prepare our data for a chi-square test: Is flake type independant from raw material type? .left-code-test-chi-square-1-auto[ ```r library(tidyverse) # filter the data to # focus on our question j_data %>% filter(Material %in% c("Volcanic", "Quartzite")) %>% filter(Artclas %in% c("Flake", "Hshat", "FFrag")) # plot the data ggplot(j_data1) + * aes(Material, * fill = Artclas) ``` ] .right-output-test-chi-square-1-auto[ ``` # A tibble: 297 x 48 Site Square Spit Group Artno Material Colour Weight Length Artclas Cortex Cortype Initiat Breaks Noseg <chr> <chr> <dbl> <dbl> <dbl> <chr> <chr> <dbl> <dbl> <chr> <dbl> <chr> <chr> <chr> <dbl> 1 J B 1 1 33 Volcanic Brown 0.23 8.5 Flake 0 <NA> Bending <NA> NA 2 J B 1 1 38 Volcanic Dk Gr… 0.06 4.24 Flake 60 Angul Hertz <NA> NA 3 J B 1 1 70 Volcanic Dk Br… 0.31 7.43 Hshat NA <NA> <NA> <NA> NA 4 J B 1 1 71 Volcanic Dk Br… 0.26 6.4 Hshat NA <NA> <NA> <NA> NA 5 J B 1 1 72 Volcanic Dk Br… 0.06 7.3 Hshat NA <NA> <NA> <NA> NA 6 J B 1 1 73 Volcanic Dk Br… 0.16 7.84 Hshat NA <NA> <NA> <NA> NA 7 J B 1 1 78 Volcanic Lt Br… 0.02 6.47 Hshat NA <NA> <NA> <NA> NA 8 J B 2 1 88 Volcanic Grey 0.38 13.3 Flake 40 Angul Hertz <NA> NA 9 J B 3 1 130 Volcanic Grey 17.8 38.4 Flake 0 <NA> Hertz <NA> NA 10 J B 3 1 131 Volcanic Grey 32.1 57.4 Flake 40 Round <NA> <NA> NA # … with 287 more rows, and 33 more variables: Platwid <dbl>, Plat <chr>, Focal <lgl>, Overhang <chr>, # NoDS <dbl>, NoPS <dbl>, Rtch <chr>, Plat_2 <chr>, Focal_2 <lgl>, Overhang_2 <chr>, NoDS_2 <dbl>, # NoPS_2 <dbl>, Rtch_2 <chr>, RetOri <chr>, Retype <chr>, Retloc <chr>, Retlen <dbl>, Retdep <dbl>, # Portion <chr>, Heat <dbl>, EdgeDam <dbl>, Weathering <dbl>, Corerot <dbl>, RetScNo <dbl>, Term <chr>, # Janus <lgl>, Recycled <lgl>, Redirect <lgl>, Width <dbl>, Thick <dbl>, Platthic <dbl>, area <dbl>, # elongation <dbl> ``` <img src="stat-inference-and-exploration-for-archaeologists_files/figure-html/test-chi-square-1_auto_6_output-1.png" width="432" /> ] --- class: split-40 count: false Prepare our data for a chi-square test: Is flake type independant from raw material type? .left-code-test-chi-square-1-auto[ ```r library(tidyverse) # filter the data to # focus on our question j_data %>% filter(Material %in% c("Volcanic", "Quartzite")) %>% filter(Artclas %in% c("Flake", "Hshat", "FFrag")) # plot the data ggplot(j_data1) + aes(Material, fill = Artclas) + * geom_bar() ``` ] .right-output-test-chi-square-1-auto[ ``` # A tibble: 297 x 48 Site Square Spit Group Artno Material Colour Weight Length Artclas Cortex Cortype Initiat Breaks Noseg <chr> <chr> <dbl> <dbl> <dbl> <chr> <chr> <dbl> <dbl> <chr> <dbl> <chr> <chr> <chr> <dbl> 1 J B 1 1 33 Volcanic Brown 0.23 8.5 Flake 0 <NA> Bending <NA> NA 2 J B 1 1 38 Volcanic Dk Gr… 0.06 4.24 Flake 60 Angul Hertz <NA> NA 3 J B 1 1 70 Volcanic Dk Br… 0.31 7.43 Hshat NA <NA> <NA> <NA> NA 4 J B 1 1 71 Volcanic Dk Br… 0.26 6.4 Hshat NA <NA> <NA> <NA> NA 5 J B 1 1 72 Volcanic Dk Br… 0.06 7.3 Hshat NA <NA> <NA> <NA> NA 6 J B 1 1 73 Volcanic Dk Br… 0.16 7.84 Hshat NA <NA> <NA> <NA> NA 7 J B 1 1 78 Volcanic Lt Br… 0.02 6.47 Hshat NA <NA> <NA> <NA> NA 8 J B 2 1 88 Volcanic Grey 0.38 13.3 Flake 40 Angul Hertz <NA> NA 9 J B 3 1 130 Volcanic Grey 17.8 38.4 Flake 0 <NA> Hertz <NA> NA 10 J B 3 1 131 Volcanic Grey 32.1 57.4 Flake 40 Round <NA> <NA> NA # … with 287 more rows, and 33 more variables: Platwid <dbl>, Plat <chr>, Focal <lgl>, Overhang <chr>, # NoDS <dbl>, NoPS <dbl>, Rtch <chr>, Plat_2 <chr>, Focal_2 <lgl>, Overhang_2 <chr>, NoDS_2 <dbl>, # NoPS_2 <dbl>, Rtch_2 <chr>, RetOri <chr>, Retype <chr>, Retloc <chr>, Retlen <dbl>, Retdep <dbl>, # Portion <chr>, Heat <dbl>, EdgeDam <dbl>, Weathering <dbl>, Corerot <dbl>, RetScNo <dbl>, Term <chr>, # Janus <lgl>, Recycled <lgl>, Redirect <lgl>, Width <dbl>, Thick <dbl>, Platthic <dbl>, area <dbl>, # elongation <dbl> ``` <img src="stat-inference-and-exploration-for-archaeologists_files/figure-html/test-chi-square-1_auto_7_output-1.png" width="432" /> ] --- class: split-40 count: false Prepare our data for a chi-square test: Is flake type independant from raw material type? .left-code-test-chi-square-1-auto[ ```r library(tidyverse) # filter the data to # focus on our question j_data %>% filter(Material %in% c("Volcanic", "Quartzite")) %>% filter(Artclas %in% c("Flake", "Hshat", "FFrag")) # plot the data ggplot(j_data1) + aes(Material, fill = Artclas) + geom_bar() + * theme_bw(base_size = 20) ``` ] .right-output-test-chi-square-1-auto[ ``` # A tibble: 297 x 48 Site Square Spit Group Artno Material Colour Weight Length Artclas Cortex Cortype Initiat Breaks Noseg <chr> <chr> <dbl> <dbl> <dbl> <chr> <chr> <dbl> <dbl> <chr> <dbl> <chr> <chr> <chr> <dbl> 1 J B 1 1 33 Volcanic Brown 0.23 8.5 Flake 0 <NA> Bending <NA> NA 2 J B 1 1 38 Volcanic Dk Gr… 0.06 4.24 Flake 60 Angul Hertz <NA> NA 3 J B 1 1 70 Volcanic Dk Br… 0.31 7.43 Hshat NA <NA> <NA> <NA> NA 4 J B 1 1 71 Volcanic Dk Br… 0.26 6.4 Hshat NA <NA> <NA> <NA> NA 5 J B 1 1 72 Volcanic Dk Br… 0.06 7.3 Hshat NA <NA> <NA> <NA> NA 6 J B 1 1 73 Volcanic Dk Br… 0.16 7.84 Hshat NA <NA> <NA> <NA> NA 7 J B 1 1 78 Volcanic Lt Br… 0.02 6.47 Hshat NA <NA> <NA> <NA> NA 8 J B 2 1 88 Volcanic Grey 0.38 13.3 Flake 40 Angul Hertz <NA> NA 9 J B 3 1 130 Volcanic Grey 17.8 38.4 Flake 0 <NA> Hertz <NA> NA 10 J B 3 1 131 Volcanic Grey 32.1 57.4 Flake 40 Round <NA> <NA> NA # … with 287 more rows, and 33 more variables: Platwid <dbl>, Plat <chr>, Focal <lgl>, Overhang <chr>, # NoDS <dbl>, NoPS <dbl>, Rtch <chr>, Plat_2 <chr>, Focal_2 <lgl>, Overhang_2 <chr>, NoDS_2 <dbl>, # NoPS_2 <dbl>, Rtch_2 <chr>, RetOri <chr>, Retype <chr>, Retloc <chr>, Retlen <dbl>, Retdep <dbl>, # Portion <chr>, Heat <dbl>, EdgeDam <dbl>, Weathering <dbl>, Corerot <dbl>, RetScNo <dbl>, Term <chr>, # Janus <lgl>, Recycled <lgl>, Redirect <lgl>, Width <dbl>, Thick <dbl>, Platthic <dbl>, area <dbl>, # elongation <dbl> ``` <img src="stat-inference-and-exploration-for-archaeologists_files/figure-html/test-chi-square-1_auto_8_output-1.png" width="432" /> ] --- class: split-40 count: false Prepare our data for a chi-square test: Is flake type independant from raw material type? .left-code-test-chi-square-1-auto[ ```r library(tidyverse) # filter the data to # focus on our question j_data %>% filter(Material %in% c("Volcanic", "Quartzite")) %>% filter(Artclas %in% c("Flake", "Hshat", "FFrag")) # plot the data ggplot(j_data1) + aes(Material, fill = Artclas) + geom_bar() + theme_bw(base_size = 20) ``` ] .right-output-test-chi-square-1-auto[ ``` # A tibble: 297 x 48 Site Square Spit Group Artno Material Colour Weight Length Artclas Cortex Cortype Initiat Breaks Noseg <chr> <chr> <dbl> <dbl> <dbl> <chr> <chr> <dbl> <dbl> <chr> <dbl> <chr> <chr> <chr> <dbl> 1 J B 1 1 33 Volcanic Brown 0.23 8.5 Flake 0 <NA> Bending <NA> NA 2 J B 1 1 38 Volcanic Dk Gr… 0.06 4.24 Flake 60 Angul Hertz <NA> NA 3 J B 1 1 70 Volcanic Dk Br… 0.31 7.43 Hshat NA <NA> <NA> <NA> NA 4 J B 1 1 71 Volcanic Dk Br… 0.26 6.4 Hshat NA <NA> <NA> <NA> NA 5 J B 1 1 72 Volcanic Dk Br… 0.06 7.3 Hshat NA <NA> <NA> <NA> NA 6 J B 1 1 73 Volcanic Dk Br… 0.16 7.84 Hshat NA <NA> <NA> <NA> NA 7 J B 1 1 78 Volcanic Lt Br… 0.02 6.47 Hshat NA <NA> <NA> <NA> NA 8 J B 2 1 88 Volcanic Grey 0.38 13.3 Flake 40 Angul Hertz <NA> NA 9 J B 3 1 130 Volcanic Grey 17.8 38.4 Flake 0 <NA> Hertz <NA> NA 10 J B 3 1 131 Volcanic Grey 32.1 57.4 Flake 40 Round <NA> <NA> NA # … with 287 more rows, and 33 more variables: Platwid <dbl>, Plat <chr>, Focal <lgl>, Overhang <chr>, # NoDS <dbl>, NoPS <dbl>, Rtch <chr>, Plat_2 <chr>, Focal_2 <lgl>, Overhang_2 <chr>, NoDS_2 <dbl>, # NoPS_2 <dbl>, Rtch_2 <chr>, RetOri <chr>, Retype <chr>, Retloc <chr>, Retlen <dbl>, Retdep <dbl>, # Portion <chr>, Heat <dbl>, EdgeDam <dbl>, Weathering <dbl>, Corerot <dbl>, RetScNo <dbl>, Term <chr>, # Janus <lgl>, Recycled <lgl>, Redirect <lgl>, Width <dbl>, Thick <dbl>, Platthic <dbl>, area <dbl>, # elongation <dbl> ``` <img src="stat-inference-and-exploration-for-archaeologists_files/figure-html/test-chi-square-1_auto_9_output-1.png" width="432" /> ] <style> .left-code-test-chi-square-1-auto { color: #777; width: 70%; height: 92%; float: left; font-size: 80% } .right-output-test-chi-square-1-auto { width: 25%; float: right; padding-left: 1%; } </style> --- class: split-40 count: false Compute the observed statistic, and use our real data to generate many fake datasets based on our null hypothesis .left-code-test-chi-square-2-auto[ ```r *library(infer) ``` ] .right-output-test-chi-square-2-auto[ ] --- class: split-40 count: false Compute the observed statistic, and use our real data to generate many fake datasets based on our null hypothesis .left-code-test-chi-square-2-auto[ ```r library(infer) # compute chi-square statistic *j_data1 ``` ] .right-output-test-chi-square-2-auto[ ``` # A tibble: 297 x 48 Site Square Spit Group Artno Material Colour Weight Length Artclas Cortex Cortype Initiat Breaks Noseg <chr> <chr> <dbl> <dbl> <dbl> <chr> <chr> <dbl> <dbl> <chr> <dbl> <chr> <chr> <chr> <dbl> 1 J B 1 1 33 Volcanic Brown 0.23 8.5 Flake 0 <NA> Bending <NA> NA 2 J B 1 1 38 Volcanic Dk Gr… 0.06 4.24 Flake 60 Angul Hertz <NA> NA 3 J B 1 1 70 Volcanic Dk Br… 0.31 7.43 Hshat NA <NA> <NA> <NA> NA 4 J B 1 1 71 Volcanic Dk Br… 0.26 6.4 Hshat NA <NA> <NA> <NA> NA 5 J B 1 1 72 Volcanic Dk Br… 0.06 7.3 Hshat NA <NA> <NA> <NA> NA 6 J B 1 1 73 Volcanic Dk Br… 0.16 7.84 Hshat NA <NA> <NA> <NA> NA 7 J B 1 1 78 Volcanic Lt Br… 0.02 6.47 Hshat NA <NA> <NA> <NA> NA 8 J B 2 1 88 Volcanic Grey 0.38 13.3 Flake 40 Angul Hertz <NA> NA 9 J B 3 1 130 Volcanic Grey 17.8 38.4 Flake 0 <NA> Hertz <NA> NA 10 J B 3 1 131 Volcanic Grey 32.1 57.4 Flake 40 Round <NA> <NA> NA # … with 287 more rows, and 33 more variables: Platwid <dbl>, Plat <chr>, Focal <lgl>, Overhang <chr>, # NoDS <dbl>, NoPS <dbl>, Rtch <chr>, Plat_2 <chr>, Focal_2 <lgl>, Overhang_2 <chr>, NoDS_2 <dbl>, # NoPS_2 <dbl>, Rtch_2 <chr>, RetOri <chr>, Retype <chr>, Retloc <chr>, Retlen <dbl>, Retdep <dbl>, # Portion <chr>, Heat <dbl>, EdgeDam <dbl>, Weathering <dbl>, Corerot <dbl>, RetScNo <dbl>, Term <chr>, # Janus <lgl>, Recycled <lgl>, Redirect <lgl>, Width <dbl>, Thick <dbl>, Platthic <dbl>, area <dbl>, # elongation <dbl> ``` ] --- class: split-40 count: false Compute the observed statistic, and use our real data to generate many fake datasets based on our null hypothesis .left-code-test-chi-square-2-auto[ ```r library(infer) # compute chi-square statistic j_data1 %>% * specify(Material ~ Artclas) ``` ] .right-output-test-chi-square-2-auto[ ``` Response: Material (factor) Explanatory: Artclas (factor) # A tibble: 297 x 2 Material Artclas <fct> <fct> 1 Volcanic Flake 2 Volcanic Flake 3 Volcanic Hshat 4 Volcanic Hshat 5 Volcanic Hshat 6 Volcanic Hshat 7 Volcanic Hshat 8 Volcanic Flake 9 Volcanic Flake 10 Volcanic Flake # … with 287 more rows ``` ] --- class: split-40 count: false Compute the observed statistic, and use our real data to generate many fake datasets based on our null hypothesis .left-code-test-chi-square-2-auto[ ```r library(infer) # compute chi-square statistic j_data1 %>% specify(Material ~ Artclas) %>% * calculate(stat = "Chisq") ``` ] .right-output-test-chi-square-2-auto[ ``` # A tibble: 1 x 1 stat <dbl> 1 2.63 ``` ] --- class: split-40 count: false Compute the observed statistic, and use our real data to generate many fake datasets based on our null hypothesis .left-code-test-chi-square-2-auto[ ```r library(infer) # compute chi-square statistic j_data1 %>% specify(Material ~ Artclas) %>% calculate(stat = "Chisq") %>% * dplyr::pull() ``` ] .right-output-test-chi-square-2-auto[ ``` X-squared 2.630641 ``` ] --- class: split-40 count: false Compute the observed statistic, and use our real data to generate many fake datasets based on our null hypothesis .left-code-test-chi-square-2-auto[ ```r library(infer) # compute chi-square statistic j_data1 %>% specify(Material ~ Artclas) %>% calculate(stat = "Chisq") %>% dplyr::pull() # generate data and chi-square # statistics under the null *j_data1 ``` ] .right-output-test-chi-square-2-auto[ ``` X-squared 2.630641 ``` ``` # A tibble: 297 x 48 Site Square Spit Group Artno Material Colour Weight Length Artclas Cortex Cortype Initiat Breaks Noseg <chr> <chr> <dbl> <dbl> <dbl> <chr> <chr> <dbl> <dbl> <chr> <dbl> <chr> <chr> <chr> <dbl> 1 J B 1 1 33 Volcanic Brown 0.23 8.5 Flake 0 <NA> Bending <NA> NA 2 J B 1 1 38 Volcanic Dk Gr… 0.06 4.24 Flake 60 Angul Hertz <NA> NA 3 J B 1 1 70 Volcanic Dk Br… 0.31 7.43 Hshat NA <NA> <NA> <NA> NA 4 J B 1 1 71 Volcanic Dk Br… 0.26 6.4 Hshat NA <NA> <NA> <NA> NA 5 J B 1 1 72 Volcanic Dk Br… 0.06 7.3 Hshat NA <NA> <NA> <NA> NA 6 J B 1 1 73 Volcanic Dk Br… 0.16 7.84 Hshat NA <NA> <NA> <NA> NA 7 J B 1 1 78 Volcanic Lt Br… 0.02 6.47 Hshat NA <NA> <NA> <NA> NA 8 J B 2 1 88 Volcanic Grey 0.38 13.3 Flake 40 Angul Hertz <NA> NA 9 J B 3 1 130 Volcanic Grey 17.8 38.4 Flake 0 <NA> Hertz <NA> NA 10 J B 3 1 131 Volcanic Grey 32.1 57.4 Flake 40 Round <NA> <NA> NA # … with 287 more rows, and 33 more variables: Platwid <dbl>, Plat <chr>, Focal <lgl>, Overhang <chr>, # NoDS <dbl>, NoPS <dbl>, Rtch <chr>, Plat_2 <chr>, Focal_2 <lgl>, Overhang_2 <chr>, NoDS_2 <dbl>, # NoPS_2 <dbl>, Rtch_2 <chr>, RetOri <chr>, Retype <chr>, Retloc <chr>, Retlen <dbl>, Retdep <dbl>, # Portion <chr>, Heat <dbl>, EdgeDam <dbl>, Weathering <dbl>, Corerot <dbl>, RetScNo <dbl>, Term <chr>, # Janus <lgl>, Recycled <lgl>, Redirect <lgl>, Width <dbl>, Thick <dbl>, Platthic <dbl>, area <dbl>, # elongation <dbl> ``` ] --- class: split-40 count: false Compute the observed statistic, and use our real data to generate many fake datasets based on our null hypothesis .left-code-test-chi-square-2-auto[ ```r library(infer) # compute chi-square statistic j_data1 %>% specify(Material ~ Artclas) %>% calculate(stat = "Chisq") %>% dplyr::pull() # generate data and chi-square # statistics under the null j_data1 %>% * specify(Material ~ Artclas) ``` ] .right-output-test-chi-square-2-auto[ ``` X-squared 2.630641 ``` ``` Response: Material (factor) Explanatory: Artclas (factor) # A tibble: 297 x 2 Material Artclas <fct> <fct> 1 Volcanic Flake 2 Volcanic Flake 3 Volcanic Hshat 4 Volcanic Hshat 5 Volcanic Hshat 6 Volcanic Hshat 7 Volcanic Hshat 8 Volcanic Flake 9 Volcanic Flake 10 Volcanic Flake # … with 287 more rows ``` ] --- class: split-40 count: false Compute the observed statistic, and use our real data to generate many fake datasets based on our null hypothesis .left-code-test-chi-square-2-auto[ ```r library(infer) # compute chi-square statistic j_data1 %>% specify(Material ~ Artclas) %>% calculate(stat = "Chisq") %>% dplyr::pull() # generate data and chi-square # statistics under the null j_data1 %>% specify(Material ~ Artclas) %>% * hypothesize(null = "independence") ``` ] .right-output-test-chi-square-2-auto[ ``` X-squared 2.630641 ``` ``` Response: Material (factor) Explanatory: Artclas (factor) Null Hypothesis: independence # A tibble: 297 x 2 Material Artclas <fct> <fct> 1 Volcanic Flake 2 Volcanic Flake 3 Volcanic Hshat 4 Volcanic Hshat 5 Volcanic Hshat 6 Volcanic Hshat 7 Volcanic Hshat 8 Volcanic Flake 9 Volcanic Flake 10 Volcanic Flake # … with 287 more rows ``` ] --- class: split-40 count: false Compute the observed statistic, and use our real data to generate many fake datasets based on our null hypothesis .left-code-test-chi-square-2-auto[ ```r library(infer) # compute chi-square statistic j_data1 %>% specify(Material ~ Artclas) %>% calculate(stat = "Chisq") %>% dplyr::pull() # generate data and chi-square # statistics under the null j_data1 %>% specify(Material ~ Artclas) %>% hypothesize(null = "independence") %>% * generate(reps = 1000, * type = "permute") ``` ] .right-output-test-chi-square-2-auto[ ``` X-squared 2.630641 ``` ``` Response: Material (factor) Explanatory: Artclas (factor) Null Hypothesis: independence # A tibble: 297,000 x 3 # Groups: replicate [1,000] Material Artclas replicate <fct> <fct> <int> 1 Volcanic Flake 1 2 Volcanic Flake 1 3 Volcanic Hshat 1 4 Volcanic Hshat 1 5 Quartzite Hshat 1 6 Volcanic Hshat 1 7 Volcanic Hshat 1 8 Volcanic Flake 1 9 Volcanic Flake 1 10 Volcanic Flake 1 # … with 296,990 more rows ``` ] --- class: split-40 count: false Compute the observed statistic, and use our real data to generate many fake datasets based on our null hypothesis .left-code-test-chi-square-2-auto[ ```r library(infer) # compute chi-square statistic j_data1 %>% specify(Material ~ Artclas) %>% calculate(stat = "Chisq") %>% dplyr::pull() # generate data and chi-square # statistics under the null j_data1 %>% specify(Material ~ Artclas) %>% hypothesize(null = "independence") %>% generate(reps = 1000, type = "permute") %>% * calculate(stat = "Chisq") ``` ] .right-output-test-chi-square-2-auto[ ``` X-squared 2.630641 ``` ``` # A tibble: 1,000 x 2 replicate stat <int> <dbl> 1 1 2.63 2 2 0.581 3 3 1.28 4 4 3.82 5 5 0.729 6 6 0.729 7 7 0.729 8 8 3.84 9 9 2.00 10 10 1.28 # … with 990 more rows ``` ] <style> .left-code-test-chi-square-2-auto { color: #777; width: 55%; height: 92%; float: left; font-size: 80% } .right-output-test-chi-square-2-auto { width: 40%; float: right; padding-left: 1%; } </style> --- class: left, top background-image: url(https://images.unsplash.com/photo-1557318041-1ce374d55ebf?ixlib=rb-1.2.1&ixid=eyJhcHBfaWQiOjEyMDd9&auto=format&fit=crop&w=1100&q=80) background-size: cover # How does our actual chi-square value for our data compare to the chi-square values we got from the datasets generated under the null hypothesis? --- class: split-40 count: false Visualise the chi-square test for our data with the random data .left-code-test-chi-square-3-auto[ ```r # visualise *null_distribution ``` ] .right-output-test-chi-square-3-auto[ ``` # A tibble: 1,000 x 2 replicate stat <int> <dbl> 1 1 6.47 2 2 2.00 3 3 0.729 4 4 2.63 5 5 7.04 6 6 0.729 7 7 3.84 8 8 1.28 9 9 0.729 10 10 0.971 # … with 990 more rows ``` ] --- class: split-40 count: false Visualise the chi-square test for our data with the random data .left-code-test-chi-square-3-auto[ ```r # visualise null_distribution %>% * visualize() ``` ] .right-output-test-chi-square-3-auto[ <img src="stat-inference-and-exploration-for-archaeologists_files/figure-html/test-chi-square-3_auto_2_output-1.png" width="432" /> ] --- class: split-40 count: false Visualise the chi-square test for our data with the random data .left-code-test-chi-square-3-auto[ ```r # visualise null_distribution %>% visualize() + * shade_p_value(obs_stat = chi_square_stat, * direction = "right") ``` ] .right-output-test-chi-square-3-auto[ <img src="stat-inference-and-exploration-for-archaeologists_files/figure-html/test-chi-square-3_auto_3_output-1.png" width="432" /> ] --- class: split-40 count: false Visualise the chi-square test for our data with the random data .left-code-test-chi-square-3-auto[ ```r # visualise null_distribution %>% visualize() + shade_p_value(obs_stat = chi_square_stat, direction = "right") + * theme_bw(base_size = 20) ``` ] .right-output-test-chi-square-3-auto[ <img src="stat-inference-and-exploration-for-archaeologists_files/figure-html/test-chi-square-3_auto_4_output-1.png" width="432" /> ] <style> .left-code-test-chi-square-3-auto { color: #777; width: 55%; height: 92%; float: left; font-size: 80% } .right-output-test-chi-square-3-auto { width: 40%; float: right; padding-left: 1%; } </style> --- class: right, top background-image: url(https://images.unsplash.com/photo-1590412732788-d54badbebb53?ixlib=rb-1.2.1&ixid=eyJhcHBfaWQiOjEyMDd9&auto=format&fit=crop&w=1651&q=80) background-size: cover # What does it mean? --- class: split-40 count: false .left-code-test-chi-square-4-auto[ ```r # get the chi-square statistic, # degrees of freedom, and p-value *j_data1 ``` ] .right-output-test-chi-square-4-auto[ ``` # A tibble: 297 x 48 Site Square Spit Group Artno Material Colour Weight Length Artclas Cortex Cortype Initiat Breaks Noseg <chr> <chr> <dbl> <dbl> <dbl> <chr> <chr> <dbl> <dbl> <chr> <dbl> <chr> <chr> <chr> <dbl> 1 J B 1 1 33 Volcanic Brown 0.23 8.5 Flake 0 <NA> Bending <NA> NA 2 J B 1 1 38 Volcanic Dk Gr… 0.06 4.24 Flake 60 Angul Hertz <NA> NA 3 J B 1 1 70 Volcanic Dk Br… 0.31 7.43 Hshat NA <NA> <NA> <NA> NA 4 J B 1 1 71 Volcanic Dk Br… 0.26 6.4 Hshat NA <NA> <NA> <NA> NA 5 J B 1 1 72 Volcanic Dk Br… 0.06 7.3 Hshat NA <NA> <NA> <NA> NA 6 J B 1 1 73 Volcanic Dk Br… 0.16 7.84 Hshat NA <NA> <NA> <NA> NA 7 J B 1 1 78 Volcanic Lt Br… 0.02 6.47 Hshat NA <NA> <NA> <NA> NA 8 J B 2 1 88 Volcanic Grey 0.38 13.3 Flake 40 Angul Hertz <NA> NA 9 J B 3 1 130 Volcanic Grey 17.8 38.4 Flake 0 <NA> Hertz <NA> NA 10 J B 3 1 131 Volcanic Grey 32.1 57.4 Flake 40 Round <NA> <NA> NA # … with 287 more rows, and 33 more variables: Platwid <dbl>, Plat <chr>, Focal <lgl>, Overhang <chr>, # NoDS <dbl>, NoPS <dbl>, Rtch <chr>, Plat_2 <chr>, Focal_2 <lgl>, Overhang_2 <chr>, NoDS_2 <dbl>, # NoPS_2 <dbl>, Rtch_2 <chr>, RetOri <chr>, Retype <chr>, Retloc <chr>, Retlen <dbl>, Retdep <dbl>, # Portion <chr>, Heat <dbl>, EdgeDam <dbl>, Weathering <dbl>, Corerot <dbl>, RetScNo <dbl>, Term <chr>, # Janus <lgl>, Recycled <lgl>, Redirect <lgl>, Width <dbl>, Thick <dbl>, Platthic <dbl>, area <dbl>, # elongation <dbl> ``` ] --- class: split-40 count: false .left-code-test-chi-square-4-auto[ ```r # get the chi-square statistic, # degrees of freedom, and p-value j_data1 %>% * chisq_test(Material ~ Artclas) ``` ] .right-output-test-chi-square-4-auto[ ``` # A tibble: 1 x 3 statistic chisq_df p_value <dbl> <int> <dbl> 1 2.63 2 0.268 ``` ] <style> .left-code-test-chi-square-4-auto { color: #777; width: 55%; height: 92%; float: left; font-size: 80% } .right-output-test-chi-square-4-auto { width: 40%; float: right; padding-left: 1%; } </style> --- # How to report the results of our chi-square test? "A chi-square test of independence showed that there was no association between raw material and artefact type (χ<sup>2</sup> (df = 2, N = 297) = 2.63, p = 0.28)." --- # Your turn: chi-square ```r library(tidyverse); library(infer) # filter the data to focus on our question j_data1 <- j_data %>% filter(Material %in% c("Volcanic", "Quartzite")) %>% filter(Artclas %in% c("Flake", "Hshat", "FFrag")) ``` ```r null_distribution <- j_data1 %>% specify(Material ~ Artclas) %>% hypothesize(null = "independence") %>% generate(reps = 1000, type = "permute") %>% calculate(stat = "Chisq") ``` ```r # visualise null_distribution %>% visualize() + shade_p_value(obs_stat = chi_square_stat, direction = "right") + theme_bw(base_size = 20) ```
07
:
00
--- # Let's do a t-test for the independence of flake length by two types of raw material in our stone artefacts. -- # Are the lengths of Volcanic and Chert flakes equivalent or different? --- class: center, left .pull-left[ 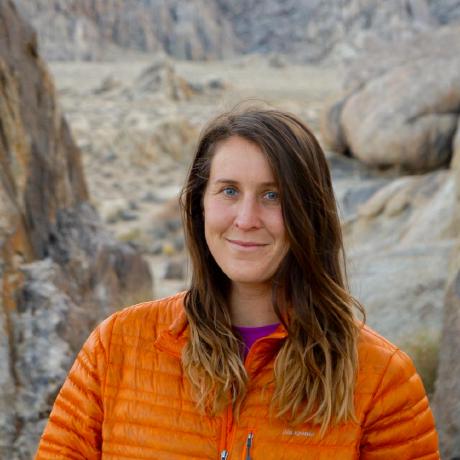 ] .pull-right[ # Illustations by Allison Horst Artist-in-Residence at RStudio ] --- class: left, bottom background-image: url(figures/allison-horst-t-test-1.jpeg) background-size: contain --- class: left, bottom background-image: url(figures/allison-horst-t-test-2.jpeg) background-size: contain --- class: left, bottom background-image: url(figures/allison-horst-t-test-3.jpeg) background-size: contain --- class: left, bottom background-image: url(figures/allison-horst-t-test-4.jpeg) background-size: contain --- class: left, bottom background-image: url(figures/allison-horst-t-test-5.jpeg) background-size: contain --- class: left, bottom background-image: url(figures/allison-horst-t-test-6.jpeg) background-size: contain --- class: left, bottom background-image: url(figures/allison-horst-t-test-7.jpeg) background-size: contain --- class: split-40 count: false Prepare our data for a t-test: Is flake length independant from raw material type? .left-code-t-test-1-auto[ ```r # filter the data to # focus on our question # prepare data *j_data2 <- j_data ``` ] .right-output-t-test-1-auto[ ] --- class: split-40 count: false Prepare our data for a t-test: Is flake length independant from raw material type? .left-code-t-test-1-auto[ ```r # filter the data to # focus on our question # prepare data j_data2 <- j_data %>% * filter(Material %in% c("Volcanic", "Chert")) ``` ] .right-output-t-test-1-auto[ ] --- class: split-40 count: false Prepare our data for a t-test: Is flake length independant from raw material type? .left-code-t-test-1-auto[ ```r # filter the data to # focus on our question # prepare data j_data2 <- j_data %>% filter(Material %in% c("Volcanic", "Chert")) %>% * filter(Artclas == "Flake") ``` ] .right-output-t-test-1-auto[ ] --- class: split-40 count: false Prepare our data for a t-test: Is flake length independant from raw material type? .left-code-t-test-1-auto[ ```r # filter the data to # focus on our question # prepare data j_data2 <- j_data %>% filter(Material %in% c("Volcanic", "Chert")) %>% filter(Artclas == "Flake") # plot the data *ggplot(j_data2) ``` ] .right-output-t-test-1-auto[ <img src="stat-inference-and-exploration-for-archaeologists_files/figure-html/t-test-1_auto_4_output-1.png" width="432" /> ] --- class: split-40 count: false Prepare our data for a t-test: Is flake length independant from raw material type? .left-code-t-test-1-auto[ ```r # filter the data to # focus on our question # prepare data j_data2 <- j_data %>% filter(Material %in% c("Volcanic", "Chert")) %>% filter(Artclas == "Flake") # plot the data ggplot(j_data2) + * aes(x = Length, * colour = Material) ``` ] .right-output-t-test-1-auto[ <img src="stat-inference-and-exploration-for-archaeologists_files/figure-html/t-test-1_auto_5_output-1.png" width="432" /> ] --- class: split-40 count: false Prepare our data for a t-test: Is flake length independant from raw material type? .left-code-t-test-1-auto[ ```r # filter the data to # focus on our question # prepare data j_data2 <- j_data %>% filter(Material %in% c("Volcanic", "Chert")) %>% filter(Artclas == "Flake") # plot the data ggplot(j_data2) + aes(x = Length, colour = Material) + * geom_density() ``` ] .right-output-t-test-1-auto[ <img src="stat-inference-and-exploration-for-archaeologists_files/figure-html/t-test-1_auto_6_output-1.png" width="432" /> ] --- class: split-40 count: false Prepare our data for a t-test: Is flake length independant from raw material type? .left-code-t-test-1-auto[ ```r # filter the data to # focus on our question # prepare data j_data2 <- j_data %>% filter(Material %in% c("Volcanic", "Chert")) %>% filter(Artclas == "Flake") # plot the data ggplot(j_data2) + aes(x = Length, colour = Material) + geom_density() + * theme_bw(base_size = 20) ``` ] .right-output-t-test-1-auto[ <img src="stat-inference-and-exploration-for-archaeologists_files/figure-html/t-test-1_auto_7_output-1.png" width="432" /> ] --- class: split-40 count: false Prepare our data for a t-test: Is flake length independant from raw material type? .left-code-t-test-1-auto[ ```r # filter the data to # focus on our question # prepare data j_data2 <- j_data %>% filter(Material %in% c("Volcanic", "Chert")) %>% filter(Artclas == "Flake") # plot the data ggplot(j_data2) + aes(x = Length, colour = Material) + geom_density() + theme_bw(base_size = 20) ``` ] .right-output-t-test-1-auto[ <img src="stat-inference-and-exploration-for-archaeologists_files/figure-html/t-test-1_auto_8_output-1.png" width="432" /> ] <style> .left-code-t-test-1-auto { color: #777; width: 60%; height: 92%; float: left; font-size: 80% } .right-output-t-test-1-auto { width: 35%; float: right; padding-left: 1%; } </style> --- class: split-40 count: false Compute the observed statistic, and use our real data to generate many fake datasets based on our null hypothesis .left-code-t-test-2b-auto[ ```r # compute t-test statistic *j_data2 ``` ] .right-output-t-test-2b-auto[ ``` # A tibble: 4,697 x 48 Site Square Spit Group Artno Material Colour Weight Length Artclas Cortex Cortype Initiat Breaks Noseg <chr> <chr> <dbl> <dbl> <dbl> <chr> <chr> <dbl> <dbl> <chr> <dbl> <chr> <chr> <chr> <dbl> 1 J B 1 1 1 Chert Brown 1.83 22.0 Flake 0 <NA> Hertz <NA> NA 2 J B 1 1 5 Chert Grey 0.05 7.07 Flake 0 <NA> Hertz <NA> NA 3 J B 1 1 7 Chert Dk Br… 0.03 5.72 Flake 0 <NA> Hertz <NA> NA 4 J B 1 1 10 Chert Grey 0.25 14.3 Flake 0 <NA> Hertz <NA> NA 5 J B 1 1 11 Chert Brown 0.33 11.2 Flake 0 <NA> Hertz <NA> NA 6 J B 1 1 18 Chert Lt Br… 0.03 3.58 Flake 0 <NA> Hertz <NA> NA 7 J B 1 1 19 Chert Red 0.02 4.79 Flake 0 <NA> Hertz Trans NA 8 J B 1 1 20 Chert Grey 0.01 3.29 Flake 0 <NA> Hertz <NA> NA 9 J B 1 1 21 Chert Brown 0.06 6.02 Flake 0 <NA> Hertz <NA> NA 10 J B 1 1 22 Chert Pink 0.01 3.51 Flake 0 <NA> Hertz <NA> NA # … with 4,687 more rows, and 33 more variables: Platwid <dbl>, Plat <chr>, Focal <lgl>, Overhang <chr>, # NoDS <dbl>, NoPS <dbl>, Rtch <chr>, Plat_2 <chr>, Focal_2 <lgl>, Overhang_2 <chr>, NoDS_2 <dbl>, # NoPS_2 <dbl>, Rtch_2 <chr>, RetOri <chr>, Retype <chr>, Retloc <chr>, Retlen <dbl>, Retdep <dbl>, # Portion <chr>, Heat <dbl>, EdgeDam <dbl>, Weathering <dbl>, Corerot <dbl>, RetScNo <dbl>, Term <chr>, # Janus <lgl>, Recycled <lgl>, Redirect <lgl>, Width <dbl>, Thick <dbl>, Platthic <dbl>, area <dbl>, # elongation <dbl> ``` ] --- class: split-40 count: false Compute the observed statistic, and use our real data to generate many fake datasets based on our null hypothesis .left-code-t-test-2b-auto[ ```r # compute t-test statistic j_data2 %>% * specify(Length ~ Material) ``` ] .right-output-t-test-2b-auto[ ``` Response: Length (numeric) Explanatory: Material (factor) # A tibble: 4,697 x 2 Length Material <dbl> <fct> 1 22.0 Chert 2 7.07 Chert 3 5.72 Chert 4 14.3 Chert 5 11.2 Chert 6 3.58 Chert 7 4.79 Chert 8 3.29 Chert 9 6.02 Chert 10 3.51 Chert # … with 4,687 more rows ``` ] --- class: split-40 count: false Compute the observed statistic, and use our real data to generate many fake datasets based on our null hypothesis .left-code-t-test-2b-auto[ ```r # compute t-test statistic j_data2 %>% specify(Length ~ Material) %>% * calculate(stat = "t", * order = c("Volcanic", "Chert")) ``` ] .right-output-t-test-2b-auto[ ``` # A tibble: 1 x 1 stat <dbl> 1 6.72 ``` ] --- class: split-40 count: false Compute the observed statistic, and use our real data to generate many fake datasets based on our null hypothesis .left-code-t-test-2b-auto[ ```r # compute t-test statistic j_data2 %>% specify(Length ~ Material) %>% calculate(stat = "t", order = c("Volcanic", "Chert")) # generate data and t-test # statistics under the null *j_data2 ``` ] .right-output-t-test-2b-auto[ ``` # A tibble: 1 x 1 stat <dbl> 1 6.72 ``` ``` # A tibble: 4,697 x 48 Site Square Spit Group Artno Material Colour Weight Length Artclas Cortex Cortype Initiat Breaks Noseg <chr> <chr> <dbl> <dbl> <dbl> <chr> <chr> <dbl> <dbl> <chr> <dbl> <chr> <chr> <chr> <dbl> 1 J B 1 1 1 Chert Brown 1.83 22.0 Flake 0 <NA> Hertz <NA> NA 2 J B 1 1 5 Chert Grey 0.05 7.07 Flake 0 <NA> Hertz <NA> NA 3 J B 1 1 7 Chert Dk Br… 0.03 5.72 Flake 0 <NA> Hertz <NA> NA 4 J B 1 1 10 Chert Grey 0.25 14.3 Flake 0 <NA> Hertz <NA> NA 5 J B 1 1 11 Chert Brown 0.33 11.2 Flake 0 <NA> Hertz <NA> NA 6 J B 1 1 18 Chert Lt Br… 0.03 3.58 Flake 0 <NA> Hertz <NA> NA 7 J B 1 1 19 Chert Red 0.02 4.79 Flake 0 <NA> Hertz Trans NA 8 J B 1 1 20 Chert Grey 0.01 3.29 Flake 0 <NA> Hertz <NA> NA 9 J B 1 1 21 Chert Brown 0.06 6.02 Flake 0 <NA> Hertz <NA> NA 10 J B 1 1 22 Chert Pink 0.01 3.51 Flake 0 <NA> Hertz <NA> NA # … with 4,687 more rows, and 33 more variables: Platwid <dbl>, Plat <chr>, Focal <lgl>, Overhang <chr>, # NoDS <dbl>, NoPS <dbl>, Rtch <chr>, Plat_2 <chr>, Focal_2 <lgl>, Overhang_2 <chr>, NoDS_2 <dbl>, # NoPS_2 <dbl>, Rtch_2 <chr>, RetOri <chr>, Retype <chr>, Retloc <chr>, Retlen <dbl>, Retdep <dbl>, # Portion <chr>, Heat <dbl>, EdgeDam <dbl>, Weathering <dbl>, Corerot <dbl>, RetScNo <dbl>, Term <chr>, # Janus <lgl>, Recycled <lgl>, Redirect <lgl>, Width <dbl>, Thick <dbl>, Platthic <dbl>, area <dbl>, # elongation <dbl> ``` ] --- class: split-40 count: false Compute the observed statistic, and use our real data to generate many fake datasets based on our null hypothesis .left-code-t-test-2b-auto[ ```r # compute t-test statistic j_data2 %>% specify(Length ~ Material) %>% calculate(stat = "t", order = c("Volcanic", "Chert")) # generate data and t-test # statistics under the null j_data2 %>% * specify(Length ~ Material) ``` ] .right-output-t-test-2b-auto[ ``` # A tibble: 1 x 1 stat <dbl> 1 6.72 ``` ``` Response: Length (numeric) Explanatory: Material (factor) # A tibble: 4,697 x 2 Length Material <dbl> <fct> 1 22.0 Chert 2 7.07 Chert 3 5.72 Chert 4 14.3 Chert 5 11.2 Chert 6 3.58 Chert 7 4.79 Chert 8 3.29 Chert 9 6.02 Chert 10 3.51 Chert # … with 4,687 more rows ``` ] --- class: split-40 count: false Compute the observed statistic, and use our real data to generate many fake datasets based on our null hypothesis .left-code-t-test-2b-auto[ ```r # compute t-test statistic j_data2 %>% specify(Length ~ Material) %>% calculate(stat = "t", order = c("Volcanic", "Chert")) # generate data and t-test # statistics under the null j_data2 %>% specify(Length ~ Material) %>% * hypothesize(null = "independence") ``` ] .right-output-t-test-2b-auto[ ``` # A tibble: 1 x 1 stat <dbl> 1 6.72 ``` ``` Response: Length (numeric) Explanatory: Material (factor) Null Hypothesis: independence # A tibble: 4,697 x 2 Length Material <dbl> <fct> 1 22.0 Chert 2 7.07 Chert 3 5.72 Chert 4 14.3 Chert 5 11.2 Chert 6 3.58 Chert 7 4.79 Chert 8 3.29 Chert 9 6.02 Chert 10 3.51 Chert # … with 4,687 more rows ``` ] --- class: split-40 count: false Compute the observed statistic, and use our real data to generate many fake datasets based on our null hypothesis .left-code-t-test-2b-auto[ ```r # compute t-test statistic j_data2 %>% specify(Length ~ Material) %>% calculate(stat = "t", order = c("Volcanic", "Chert")) # generate data and t-test # statistics under the null j_data2 %>% specify(Length ~ Material) %>% hypothesize(null = "independence") %>% * generate(reps = 1000, * type = "permute") ``` ] .right-output-t-test-2b-auto[ ``` # A tibble: 1 x 1 stat <dbl> 1 6.72 ``` ``` Response: Length (numeric) Explanatory: Material (factor) Null Hypothesis: independence # A tibble: 4,697,000 x 3 # Groups: replicate [1,000] Length Material replicate <dbl> <fct> <int> 1 9.36 Chert 1 2 7.14 Chert 1 3 22.1 Chert 1 4 5.22 Chert 1 5 21.1 Chert 1 6 6.08 Chert 1 7 10.7 Chert 1 8 10.7 Chert 1 9 3.88 Chert 1 10 11.6 Chert 1 # … with 4,696,990 more rows ``` ] --- class: split-40 count: false Compute the observed statistic, and use our real data to generate many fake datasets based on our null hypothesis .left-code-t-test-2b-auto[ ```r # compute t-test statistic j_data2 %>% specify(Length ~ Material) %>% calculate(stat = "t", order = c("Volcanic", "Chert")) # generate data and t-test # statistics under the null j_data2 %>% specify(Length ~ Material) %>% hypothesize(null = "independence") %>% generate(reps = 1000, type = "permute") %>% * calculate(stat = "t", * order = c("Volcanic", "Chert")) ``` ] .right-output-t-test-2b-auto[ ``` # A tibble: 1 x 1 stat <dbl> 1 6.72 ``` ``` # A tibble: 1,000 x 2 replicate stat <int> <dbl> 1 1 -0.436 2 2 -1.08 3 3 1.47 4 4 -0.744 5 5 0.309 6 6 1.37 7 7 0.258 8 8 -2.28 9 9 -2.04 10 10 0.766 # … with 990 more rows ``` ] <style> .left-code-t-test-2b-auto { color: #777; width: 55%; height: 92%; float: left; font-size: 80% } .right-output-t-test-2b-auto { width: 40%; float: right; padding-left: 1%; } </style> --- class: split-40 count: false Visualise the t-test for our data with the random data .left-code-t-test-3-auto[ ```r # visualise *null_distribution ``` ] .right-output-t-test-3-auto[ ``` # A tibble: 1,000 x 2 replicate stat <int> <dbl> 1 1 1.18 2 2 0.232 3 3 0.790 4 4 -0.966 5 5 -1.12 6 6 -0.429 7 7 -0.125 8 8 1.95 9 9 -0.105 10 10 0.299 # … with 990 more rows ``` ] --- class: split-40 count: false Visualise the t-test for our data with the random data .left-code-t-test-3-auto[ ```r # visualise null_distribution %>% * visualize() ``` ] .right-output-t-test-3-auto[ <img src="stat-inference-and-exploration-for-archaeologists_files/figure-html/t-test-3_auto_2_output-1.png" width="432" /> ] --- class: split-40 count: false Visualise the t-test for our data with the random data .left-code-t-test-3-auto[ ```r # visualise null_distribution %>% visualize() + * shade_p_value(obs_stat = t_stat, * direction = "right") ``` ] .right-output-t-test-3-auto[ <img src="stat-inference-and-exploration-for-archaeologists_files/figure-html/t-test-3_auto_3_output-1.png" width="432" /> ] --- class: split-40 count: false Visualise the t-test for our data with the random data .left-code-t-test-3-auto[ ```r # visualise null_distribution %>% visualize() + shade_p_value(obs_stat = t_stat, direction = "right") + * theme_bw(base_size = 20) ``` ] .right-output-t-test-3-auto[ <img src="stat-inference-and-exploration-for-archaeologists_files/figure-html/t-test-3_auto_4_output-1.png" width="432" /> ] <style> .left-code-t-test-3-auto { color: #777; width: 55%; height: 92%; float: left; font-size: 80% } .right-output-t-test-3-auto { width: 40%; float: right; padding-left: 1%; } </style> --- class: right, top background-image: url(https://images.unsplash.com/photo-1590034081344-88d957c6282b?ixlib=rb-1.2.1&ixid=eyJhcHBfaWQiOjEyMDd9&auto=format&fit=crop&w=1650&q=80) background-size: cover # What does it mean? --- class: split-40 count: false .left-code-t-test-4-auto[ ```r # get the t-test statistic, # degrees of freedom, and p-value *j_data1 ``` ] .right-output-t-test-4-auto[ ``` # A tibble: 297 x 48 Site Square Spit Group Artno Material Colour Weight Length Artclas Cortex Cortype Initiat Breaks Noseg <chr> <chr> <dbl> <dbl> <dbl> <chr> <chr> <dbl> <dbl> <chr> <dbl> <chr> <chr> <chr> <dbl> 1 J B 1 1 33 Volcanic Brown 0.23 8.5 Flake 0 <NA> Bending <NA> NA 2 J B 1 1 38 Volcanic Dk Gr… 0.06 4.24 Flake 60 Angul Hertz <NA> NA 3 J B 1 1 70 Volcanic Dk Br… 0.31 7.43 Hshat NA <NA> <NA> <NA> NA 4 J B 1 1 71 Volcanic Dk Br… 0.26 6.4 Hshat NA <NA> <NA> <NA> NA 5 J B 1 1 72 Volcanic Dk Br… 0.06 7.3 Hshat NA <NA> <NA> <NA> NA 6 J B 1 1 73 Volcanic Dk Br… 0.16 7.84 Hshat NA <NA> <NA> <NA> NA 7 J B 1 1 78 Volcanic Lt Br… 0.02 6.47 Hshat NA <NA> <NA> <NA> NA 8 J B 2 1 88 Volcanic Grey 0.38 13.3 Flake 40 Angul Hertz <NA> NA 9 J B 3 1 130 Volcanic Grey 17.8 38.4 Flake 0 <NA> Hertz <NA> NA 10 J B 3 1 131 Volcanic Grey 32.1 57.4 Flake 40 Round <NA> <NA> NA # … with 287 more rows, and 33 more variables: Platwid <dbl>, Plat <chr>, Focal <lgl>, Overhang <chr>, # NoDS <dbl>, NoPS <dbl>, Rtch <chr>, Plat_2 <chr>, Focal_2 <lgl>, Overhang_2 <chr>, NoDS_2 <dbl>, # NoPS_2 <dbl>, Rtch_2 <chr>, RetOri <chr>, Retype <chr>, Retloc <chr>, Retlen <dbl>, Retdep <dbl>, # Portion <chr>, Heat <dbl>, EdgeDam <dbl>, Weathering <dbl>, Corerot <dbl>, RetScNo <dbl>, Term <chr>, # Janus <lgl>, Recycled <lgl>, Redirect <lgl>, Width <dbl>, Thick <dbl>, Platthic <dbl>, area <dbl>, # elongation <dbl> ``` ] --- class: split-40 count: false .left-code-t-test-4-auto[ ```r # get the t-test statistic, # degrees of freedom, and p-value j_data1 %>% * t_test(Length ~ Material, * order = c("Volcanic", * "Quartzite")) ``` ] .right-output-t-test-4-auto[ ``` # A tibble: 1 x 6 statistic t_df p_value alternative lower_ci upper_ci <dbl> <dbl> <dbl> <chr> <dbl> <dbl> 1 0.629 36.4 0.533 two.sided -2.60 4.95 ``` ] --- class: split-40 count: false .left-code-t-test-4-auto[ ```r # get the t-test statistic, # degrees of freedom, and p-value j_data1 %>% t_test(Length ~ Material, order = c("Volcanic", "Quartzite")) %>% * str() ``` ] .right-output-t-test-4-auto[ ``` tibble [1 × 6] (S3: tbl_df/tbl/data.frame) $ statistic : Named num 0.629 ..- attr(*, "names")= chr "t" $ t_df : Named num 36.4 ..- attr(*, "names")= chr "df" $ p_value : num 0.533 $ alternative: chr "two.sided" $ lower_ci : num -2.6 $ upper_ci : num 4.95 ``` ] <style> .left-code-t-test-4-auto { color: #777; width: 50%; height: 92%; float: left; font-size: 80% } .right-output-t-test-4-auto { width: 45%; float: right; padding-left: 1%; } </style> --- # How to report the results of our t-test? "There was no association between raw material and artefact type for Volcanic and Quartzite artefacts (t(36) = 0.62, p = 0.53)." --- # Your turn: t-test ```r # filter the data to focus on our question j_data2 <- j_data %>% filter(Material %in% c("Volcanic", "Chert")) %>% filter(Artclas == "Flake") ``` ```r null_distribution <- j_data2 %>% specify(Length ~ Material) %>% hypothesize(null = "independence") %>% generate(reps = 1000, type = "permute") %>% calculate(stat = "t", order = c("Volcanic", "Chert")) ``` ```r # visualise null_distribution %>% visualize() + shade_p_value(obs_stat = t_stat, direction = "right") + theme_bw(base_size = 20) ```
07
:
00
--- # Let's do an ANOVA for the independence of flake length by _all_ types of raw material in our stone artefacts. -- # Are the lengths of flakes equivalent or different for _all_ categories of raw material? --- class: split-40 count: false Prepare and inspect our data .left-code-anova-1-auto[ ```r # filter the data to # focus on our question *j_data3 <- j_data ``` ] .right-output-anova-1-auto[ ] --- class: split-40 count: false Prepare and inspect our data .left-code-anova-1-auto[ ```r # filter the data to # focus on our question j_data3 <- j_data %>% * filter(Artclas == "Flake") ``` ] .right-output-anova-1-auto[ ] --- class: split-40 count: false Prepare and inspect our data .left-code-anova-1-auto[ ```r # filter the data to # focus on our question j_data3 <- j_data %>% filter(Artclas == "Flake") # plot the data *ggplot(j_data3) ``` ] .right-output-anova-1-auto[ <img src="stat-inference-and-exploration-for-archaeologists_files/figure-html/anova-1_auto_3_output-1.png" width="432" /> ] --- class: split-40 count: false Prepare and inspect our data .left-code-anova-1-auto[ ```r # filter the data to # focus on our question j_data3 <- j_data %>% filter(Artclas == "Flake") # plot the data ggplot(j_data3) + * aes(reorder(Material, * Length), * Length) ``` ] .right-output-anova-1-auto[ <img src="stat-inference-and-exploration-for-archaeologists_files/figure-html/anova-1_auto_4_output-1.png" width="432" /> ] --- class: split-40 count: false Prepare and inspect our data .left-code-anova-1-auto[ ```r # filter the data to # focus on our question j_data3 <- j_data %>% filter(Artclas == "Flake") # plot the data ggplot(j_data3) + aes(reorder(Material, Length), Length) + * geom_boxplot() ``` ] .right-output-anova-1-auto[ <img src="stat-inference-and-exploration-for-archaeologists_files/figure-html/anova-1_auto_5_output-1.png" width="432" /> ] --- class: split-40 count: false Prepare and inspect our data .left-code-anova-1-auto[ ```r # filter the data to # focus on our question j_data3 <- j_data %>% filter(Artclas == "Flake") # plot the data ggplot(j_data3) + aes(reorder(Material, Length), Length) + geom_boxplot() + * coord_flip() ``` ] .right-output-anova-1-auto[ <img src="stat-inference-and-exploration-for-archaeologists_files/figure-html/anova-1_auto_6_output-1.png" width="432" /> ] --- class: split-40 count: false Prepare and inspect our data .left-code-anova-1-auto[ ```r # filter the data to # focus on our question j_data3 <- j_data %>% filter(Artclas == "Flake") # plot the data ggplot(j_data3) + aes(reorder(Material, Length), Length) + geom_boxplot() + coord_flip() + * theme_bw(base_size = 20) ``` ] .right-output-anova-1-auto[ <img src="stat-inference-and-exploration-for-archaeologists_files/figure-html/anova-1_auto_7_output-1.png" width="432" /> ] --- class: split-40 count: false Prepare and inspect our data .left-code-anova-1-auto[ ```r # filter the data to # focus on our question j_data3 <- j_data %>% filter(Artclas == "Flake") # plot the data ggplot(j_data3) + aes(reorder(Material, Length), Length) + geom_boxplot() + coord_flip() + theme_bw(base_size = 20) ``` ] .right-output-anova-1-auto[ <img src="stat-inference-and-exploration-for-archaeologists_files/figure-html/anova-1_auto_8_output-1.png" width="432" /> ] <style> .left-code-anova-1-auto { color: #777; width: 38%; height: 92%; float: left; font-size: 80% } .right-output-anova-1-auto { width: 60%; float: right; padding-left: 1%; } </style> --- class: split-40 count: false Compute the observed statistic, and use our real data to generate many fake datasets based on our null hypothesis .left-code-anova-2-auto[ ```r # compute F statistic *j_data3 ``` ] .right-output-anova-2-auto[ ``` # A tibble: 4,826 x 48 Site Square Spit Group Artno Material Colour Weight Length Artclas Cortex Cortype Initiat Breaks Noseg <chr> <chr> <dbl> <dbl> <dbl> <chr> <chr> <dbl> <dbl> <chr> <dbl> <chr> <chr> <chr> <dbl> 1 J B 1 1 1 Chert Brown 1.83 22.0 Flake 0 <NA> Hertz <NA> NA 2 J B 1 1 5 Chert Grey 0.05 7.07 Flake 0 <NA> Hertz <NA> NA 3 J B 1 1 7 Chert Dk Br… 0.03 5.72 Flake 0 <NA> Hertz <NA> NA 4 J B 1 1 10 Chert Grey 0.25 14.3 Flake 0 <NA> Hertz <NA> NA 5 J B 1 1 11 Chert Brown 0.33 11.2 Flake 0 <NA> Hertz <NA> NA 6 J B 1 1 18 Chert Lt Br… 0.03 3.58 Flake 0 <NA> Hertz <NA> NA 7 J B 1 1 19 Chert Red 0.02 4.79 Flake 0 <NA> Hertz Trans NA 8 J B 1 1 20 Chert Grey 0.01 3.29 Flake 0 <NA> Hertz <NA> NA 9 J B 1 1 21 Chert Brown 0.06 6.02 Flake 0 <NA> Hertz <NA> NA 10 J B 1 1 22 Chert Pink 0.01 3.51 Flake 0 <NA> Hertz <NA> NA # … with 4,816 more rows, and 33 more variables: Platwid <dbl>, Plat <chr>, Focal <lgl>, Overhang <chr>, # NoDS <dbl>, NoPS <dbl>, Rtch <chr>, Plat_2 <chr>, Focal_2 <lgl>, Overhang_2 <chr>, NoDS_2 <dbl>, # NoPS_2 <dbl>, Rtch_2 <chr>, RetOri <chr>, Retype <chr>, Retloc <chr>, Retlen <dbl>, Retdep <dbl>, # Portion <chr>, Heat <dbl>, EdgeDam <dbl>, Weathering <dbl>, Corerot <dbl>, RetScNo <dbl>, Term <chr>, # Janus <lgl>, Recycled <lgl>, Redirect <lgl>, Width <dbl>, Thick <dbl>, Platthic <dbl>, area <dbl>, # elongation <dbl> ``` ] --- class: split-40 count: false Compute the observed statistic, and use our real data to generate many fake datasets based on our null hypothesis .left-code-anova-2-auto[ ```r # compute F statistic j_data3 %>% * specify(Length ~ Material) ``` ] .right-output-anova-2-auto[ ``` Response: Length (numeric) Explanatory: Material (factor) # A tibble: 4,826 x 2 Length Material <dbl> <fct> 1 22.0 Chert 2 7.07 Chert 3 5.72 Chert 4 14.3 Chert 5 11.2 Chert 6 3.58 Chert 7 4.79 Chert 8 3.29 Chert 9 6.02 Chert 10 3.51 Chert # … with 4,816 more rows ``` ] --- class: split-40 count: false Compute the observed statistic, and use our real data to generate many fake datasets based on our null hypothesis .left-code-anova-2-auto[ ```r # compute F statistic j_data3 %>% specify(Length ~ Material) %>% * calculate(stat = "F") ``` ] .right-output-anova-2-auto[ ``` # A tibble: 1 x 1 stat <dbl> 1 15.1 ``` ] --- class: split-40 count: false Compute the observed statistic, and use our real data to generate many fake datasets based on our null hypothesis .left-code-anova-2-auto[ ```r # compute F statistic j_data3 %>% specify(Length ~ Material) %>% calculate(stat = "F") # generate data and t-test # statistics under the null *j_data3 ``` ] .right-output-anova-2-auto[ ``` # A tibble: 1 x 1 stat <dbl> 1 15.1 ``` ``` # A tibble: 4,826 x 48 Site Square Spit Group Artno Material Colour Weight Length Artclas Cortex Cortype Initiat Breaks Noseg <chr> <chr> <dbl> <dbl> <dbl> <chr> <chr> <dbl> <dbl> <chr> <dbl> <chr> <chr> <chr> <dbl> 1 J B 1 1 1 Chert Brown 1.83 22.0 Flake 0 <NA> Hertz <NA> NA 2 J B 1 1 5 Chert Grey 0.05 7.07 Flake 0 <NA> Hertz <NA> NA 3 J B 1 1 7 Chert Dk Br… 0.03 5.72 Flake 0 <NA> Hertz <NA> NA 4 J B 1 1 10 Chert Grey 0.25 14.3 Flake 0 <NA> Hertz <NA> NA 5 J B 1 1 11 Chert Brown 0.33 11.2 Flake 0 <NA> Hertz <NA> NA 6 J B 1 1 18 Chert Lt Br… 0.03 3.58 Flake 0 <NA> Hertz <NA> NA 7 J B 1 1 19 Chert Red 0.02 4.79 Flake 0 <NA> Hertz Trans NA 8 J B 1 1 20 Chert Grey 0.01 3.29 Flake 0 <NA> Hertz <NA> NA 9 J B 1 1 21 Chert Brown 0.06 6.02 Flake 0 <NA> Hertz <NA> NA 10 J B 1 1 22 Chert Pink 0.01 3.51 Flake 0 <NA> Hertz <NA> NA # … with 4,816 more rows, and 33 more variables: Platwid <dbl>, Plat <chr>, Focal <lgl>, Overhang <chr>, # NoDS <dbl>, NoPS <dbl>, Rtch <chr>, Plat_2 <chr>, Focal_2 <lgl>, Overhang_2 <chr>, NoDS_2 <dbl>, # NoPS_2 <dbl>, Rtch_2 <chr>, RetOri <chr>, Retype <chr>, Retloc <chr>, Retlen <dbl>, Retdep <dbl>, # Portion <chr>, Heat <dbl>, EdgeDam <dbl>, Weathering <dbl>, Corerot <dbl>, RetScNo <dbl>, Term <chr>, # Janus <lgl>, Recycled <lgl>, Redirect <lgl>, Width <dbl>, Thick <dbl>, Platthic <dbl>, area <dbl>, # elongation <dbl> ``` ] --- class: split-40 count: false Compute the observed statistic, and use our real data to generate many fake datasets based on our null hypothesis .left-code-anova-2-auto[ ```r # compute F statistic j_data3 %>% specify(Length ~ Material) %>% calculate(stat = "F") # generate data and t-test # statistics under the null j_data3 %>% * specify(Length ~ Material) ``` ] .right-output-anova-2-auto[ ``` # A tibble: 1 x 1 stat <dbl> 1 15.1 ``` ``` Response: Length (numeric) Explanatory: Material (factor) # A tibble: 4,826 x 2 Length Material <dbl> <fct> 1 22.0 Chert 2 7.07 Chert 3 5.72 Chert 4 14.3 Chert 5 11.2 Chert 6 3.58 Chert 7 4.79 Chert 8 3.29 Chert 9 6.02 Chert 10 3.51 Chert # … with 4,816 more rows ``` ] --- class: split-40 count: false Compute the observed statistic, and use our real data to generate many fake datasets based on our null hypothesis .left-code-anova-2-auto[ ```r # compute F statistic j_data3 %>% specify(Length ~ Material) %>% calculate(stat = "F") # generate data and t-test # statistics under the null j_data3 %>% specify(Length ~ Material) %>% * hypothesize(null = "independence") ``` ] .right-output-anova-2-auto[ ``` # A tibble: 1 x 1 stat <dbl> 1 15.1 ``` ``` Response: Length (numeric) Explanatory: Material (factor) Null Hypothesis: independence # A tibble: 4,826 x 2 Length Material <dbl> <fct> 1 22.0 Chert 2 7.07 Chert 3 5.72 Chert 4 14.3 Chert 5 11.2 Chert 6 3.58 Chert 7 4.79 Chert 8 3.29 Chert 9 6.02 Chert 10 3.51 Chert # … with 4,816 more rows ``` ] --- class: split-40 count: false Compute the observed statistic, and use our real data to generate many fake datasets based on our null hypothesis .left-code-anova-2-auto[ ```r # compute F statistic j_data3 %>% specify(Length ~ Material) %>% calculate(stat = "F") # generate data and t-test # statistics under the null j_data3 %>% specify(Length ~ Material) %>% hypothesize(null = "independence") %>% * generate(reps = 1000, * type = "permute") ``` ] .right-output-anova-2-auto[ ``` # A tibble: 1 x 1 stat <dbl> 1 15.1 ``` ``` Response: Length (numeric) Explanatory: Material (factor) Null Hypothesis: independence # A tibble: 4,826,000 x 3 # Groups: replicate [1,000] Length Material replicate <dbl> <fct> <int> 1 13.6 Chert 1 2 4.2 Chert 1 3 12.9 Chert 1 4 6.08 Chert 1 5 13.2 Chert 1 6 8.14 Chert 1 7 22.6 Chert 1 8 5.68 Chert 1 9 17.4 Chert 1 10 10.9 Chert 1 # … with 4,825,990 more rows ``` ] --- class: split-40 count: false Compute the observed statistic, and use our real data to generate many fake datasets based on our null hypothesis .left-code-anova-2-auto[ ```r # compute F statistic j_data3 %>% specify(Length ~ Material) %>% calculate(stat = "F") # generate data and t-test # statistics under the null j_data3 %>% specify(Length ~ Material) %>% hypothesize(null = "independence") %>% generate(reps = 1000, type = "permute") %>% * calculate(stat = "F") ``` ] .right-output-anova-2-auto[ ``` # A tibble: 1 x 1 stat <dbl> 1 15.1 ``` ``` # A tibble: 1,000 x 2 replicate stat <int> <dbl> 1 1 1.68 2 2 1.24 3 3 0.366 4 4 0.939 5 5 0.485 6 6 0.876 7 7 0.695 8 8 1.04 9 9 0.607 10 10 1.40 # … with 990 more rows ``` ] <style> .left-code-anova-2-auto { color: #777; width: 65%; height: 92%; float: left; font-size: 80% } .right-output-anova-2-auto { width: 34%; float: right; padding-left: 1%; } </style> --- class: split-40 count: false Visualise the ANOVA for our data with the random data .left-code-anova-3-auto[ ```r # visualise *null_distribution ``` ] .right-output-anova-3-auto[ ``` # A tibble: 1,000 x 2 replicate stat <int> <dbl> 1 1 1.92 2 2 0.568 3 3 0.717 4 4 0.753 5 5 1.05 6 6 1.23 7 7 0.560 8 8 1.12 9 9 0.404 10 10 1.18 # … with 990 more rows ``` ] --- class: split-40 count: false Visualise the ANOVA for our data with the random data .left-code-anova-3-auto[ ```r # visualise null_distribution %>% * visualize() ``` ] .right-output-anova-3-auto[ <img src="stat-inference-and-exploration-for-archaeologists_files/figure-html/anova-3_auto_2_output-1.png" width="432" /> ] --- class: split-40 count: false Visualise the ANOVA for our data with the random data .left-code-anova-3-auto[ ```r # visualise null_distribution %>% visualize() + * shade_p_value(obs_stat = F_stat, * direction = "right") ``` ] .right-output-anova-3-auto[ <img src="stat-inference-and-exploration-for-archaeologists_files/figure-html/anova-3_auto_3_output-1.png" width="432" /> ] --- class: split-40 count: false Visualise the ANOVA for our data with the random data .left-code-anova-3-auto[ ```r # visualise null_distribution %>% visualize() + shade_p_value(obs_stat = F_stat, direction = "right") + * theme_bw(base_size = 20) ``` ] .right-output-anova-3-auto[ <img src="stat-inference-and-exploration-for-archaeologists_files/figure-html/anova-3_auto_4_output-1.png" width="432" /> ] <style> .left-code-anova-3-auto { color: #777; width: 55%; height: 92%; float: left; font-size: 80% } .right-output-anova-3-auto { width: 40%; float: right; padding-left: 1%; } </style> --- class: split-40 count: false Inspect the ANOVA output .left-code-anova-4-auto[ ```r # get the F statistic, # degrees of freedom, and p-value *aov(Length ~ Material, * data = j_data3) ``` ] .right-output-anova-4-auto[ ``` Call: aov(formula = Length ~ Material, data = j_data3) Terms: Material Residuals Sum of Squares 5640.79 180269.35 Deg. of Freedom 10 4815 Residual standard error: 6.118751 Estimated effects may be unbalanced ``` ] --- class: split-40 count: false Inspect the ANOVA output .left-code-anova-4-auto[ ```r # get the F statistic, # degrees of freedom, and p-value aov(Length ~ Material, data = j_data3) %>% * broom::tidy() ``` ] .right-output-anova-4-auto[ ``` # A tibble: 2 x 6 term df sumsq meansq statistic p.value <chr> <dbl> <dbl> <dbl> <dbl> <dbl> 1 Material 10 5641. 564. 15.1 7.66e-27 2 Residuals 4815 180269. 37.4 NA NA ``` ] --- class: split-40 count: false Inspect the ANOVA output .left-code-anova-4-auto[ ```r # get the F statistic, # degrees of freedom, and p-value aov(Length ~ Material, data = j_data3) %>% broom::tidy() # inspect specific difference # between categories *aov(Length ~ Material, * data = j_data3) ``` ] .right-output-anova-4-auto[ ``` # A tibble: 2 x 6 term df sumsq meansq statistic p.value <chr> <dbl> <dbl> <dbl> <dbl> <dbl> 1 Material 10 5641. 564. 15.1 7.66e-27 2 Residuals 4815 180269. 37.4 NA NA ``` ``` Call: aov(formula = Length ~ Material, data = j_data3) Terms: Material Residuals Sum of Squares 5640.79 180269.35 Deg. of Freedom 10 4815 Residual standard error: 6.118751 Estimated effects may be unbalanced ``` ] --- class: split-40 count: false Inspect the ANOVA output .left-code-anova-4-auto[ ```r # get the F statistic, # degrees of freedom, and p-value aov(Length ~ Material, data = j_data3) %>% broom::tidy() # inspect specific difference # between categories aov(Length ~ Material, data = j_data3) %>% * TukeyHSD() ``` ] .right-output-anova-4-auto[ ``` # A tibble: 2 x 6 term df sumsq meansq statistic p.value <chr> <dbl> <dbl> <dbl> <dbl> <dbl> 1 Material 10 5641. 564. 15.1 7.66e-27 2 Residuals 4815 180269. 37.4 NA NA ``` ``` Tukey multiple comparisons of means 95% family-wise confidence level Fit: aov(formula = Length ~ Material, data = j_data3) $Material diff lwr upr p adj Chert-Brecchert -1.9466973 -21.6525949 17.75920033 0.9999999 Cortical-Brecchert -1.1133333 -23.8651968 21.63853014 1.0000000 Dehydchert-Brecchert 0.5100000 -27.3552281 28.37522810 1.0000000 FGS-Brecchert 9.0500000 -18.8152281 36.91522810 0.9939875 Obsidian-Brecchert 0.6654545 -19.9143803 21.24528939 1.0000000 Quartz-Brecchert -0.8588235 -21.1337551 19.41610802 1.0000000 Quartzite-Brecchert 3.0370000 -16.9923947 23.06639467 0.9999934 Silcrete-Brecchert -1.4965217 -21.4132326 18.42018909 1.0000000 Unknown-Brecchert 4.4631579 -15.7524033 24.67871909 0.9997802 Volcanic-Brecchert 2.3448918 -17.4014026 22.09118615 0.9999994 Cortical-Chert 0.8333639 -10.5463880 12.21311588 1.0000000 Dehydchert-Chert 2.4566973 -17.2492003 22.16259486 0.9999990 FGS-Chert 10.9966973 -8.7092003 30.70259486 0.7822430 Obsidian-Chert 2.6121518 -3.3360466 8.56035027 0.9451831 Quartz-Chert 1.0878737 -3.7000602 5.87580773 0.9997153 Quartzite-Chert 4.9836973 1.3742494 8.59314510 0.0004617 Silcrete-Chert 0.4501755 -2.4698986 3.37024965 0.9999922 Unknown-Chert 6.4098552 1.8799129 10.93979745 0.0002781 Volcanic-Chert 4.2915890 2.9620762 5.62110188 0.0000000 Dehydchert-Cortical 1.6233333 -21.1285301 24.37519681 1.0000000 FGS-Cortical 10.1633333 -12.5885301 32.91519681 0.9387607 Obsidian-Cortical 1.7787879 -11.0549940 14.61256972 0.9999972 Quartz-Cortical 0.2545098 -12.0844206 12.59344020 1.0000000 Quartzite-Cortical 4.1503333 -7.7808445 16.08151119 0.9896880 Silcrete-Cortical -0.3831884 -12.1242158 11.35783899 1.0000000 Unknown-Cortical 5.5764912 -6.6646390 17.81762143 0.9305883 Volcanic-Cortical 3.4582251 -7.9913380 14.90778824 0.9966652 FGS-Dehydchert 8.5400000 -19.3252281 36.40522810 0.9962406 Obsidian-Dehydchert 0.1554545 -20.4243803 20.73528939 1.0000000 Quartz-Dehydchert -1.3688235 -21.6437551 18.90610802 1.0000000 Quartzite-Dehydchert 2.5270000 -17.5023947 22.55639467 0.9999989 Silcrete-Dehydchert -2.0065217 -21.9232326 17.91018909 0.9999999 Unknown-Dehydchert 3.9531579 -16.2624033 24.16871909 0.9999275 Volcanic-Dehydchert 1.8348918 -17.9114026 21.58118615 0.9999999 Obsidian-FGS -8.3845455 -28.9643803 12.19528939 0.9669934 Quartz-FGS -9.9088235 -30.1837551 10.36610802 0.8934486 Quartzite-FGS -6.0130000 -26.0423947 14.01639467 0.9968285 Silcrete-FGS -10.5465217 -30.4632326 9.37018909 0.8332796 Unknown-FGS -4.5868421 -24.8024033 15.62871909 0.9997188 Volcanic-FGS -6.7051082 -26.4514026 13.04118615 0.9914510 Quartz-Obsidian -1.5242781 -9.1486806 6.10012446 0.9999111 Quartzite-Obsidian 2.3715455 -4.5736195 9.31671038 0.9910695 Silcrete-Obsidian -2.1619763 -8.7751476 4.45119504 0.9936637 Unknown-Obsidian 3.7977033 -3.6673874 11.26279405 0.8659341 Volcanic-Obsidian 1.6794372 -4.4012541 7.76012852 0.9984250 Quartzite-Quartz 3.8958235 -2.0856949 9.87734197 0.5792526 Silcrete-Quartz -0.6376982 -6.2303078 4.95491138 0.9999996 Unknown-Quartz 5.3219814 -1.2560750 11.90003784 0.2460217 Volcanic-Quartz 3.2037153 -1.7478555 8.15528612 0.5892819 Silcrete-Quartzite -4.5335217 -9.1574901 0.09044666 0.0604008 Unknown-Quartzite 1.4261579 -4.3509199 7.20323570 0.9994046 Volcanic-Quartzite -0.6921082 -4.5159617 3.13174524 0.9999647 Unknown-Silcrete 5.9596796 0.5862871 11.33307216 0.0157827 Volcanic-Silcrete 3.8414135 0.6601285 7.02269856 0.0048717 Volcanic-Unknown -2.1182661 -6.8208312 2.58429900 0.9353882 ``` ] --- class: split-40 count: false Inspect the ANOVA output .left-code-anova-4-auto[ ```r # get the F statistic, # degrees of freedom, and p-value aov(Length ~ Material, data = j_data3) %>% broom::tidy() # inspect specific difference # between categories aov(Length ~ Material, data = j_data3) %>% TukeyHSD() %>% * broom::tidy() ``` ] .right-output-anova-4-auto[ ``` # A tibble: 2 x 6 term df sumsq meansq statistic p.value <chr> <dbl> <dbl> <dbl> <dbl> <dbl> 1 Material 10 5641. 564. 15.1 7.66e-27 2 Residuals 4815 180269. 37.4 NA NA ``` ``` # A tibble: 55 x 6 term comparison estimate conf.low conf.high adj.p.value <chr> <chr> <dbl> <dbl> <dbl> <dbl> 1 Material Chert-Brecchert -1.95 -21.7 17.8 1.00 2 Material Cortical-Brecchert -1.11 -23.9 21.6 1.00 3 Material Dehydchert-Brecchert 0.51 -27.4 28.4 1 4 Material FGS-Brecchert 9.05 -18.8 36.9 0.994 5 Material Obsidian-Brecchert 0.665 -19.9 21.2 1.00 6 Material Quartz-Brecchert -0.859 -21.1 19.4 1.00 7 Material Quartzite-Brecchert 3.04 -17.0 23.1 1.00 8 Material Silcrete-Brecchert -1.50 -21.4 18.4 1.00 9 Material Unknown-Brecchert 4.46 -15.8 24.7 1.00 10 Material Volcanic-Brecchert 2.34 -17.4 22.1 1.00 # … with 45 more rows ``` ] --- class: split-40 count: false Inspect the ANOVA output .left-code-anova-4-auto[ ```r # get the F statistic, # degrees of freedom, and p-value aov(Length ~ Material, data = j_data3) %>% broom::tidy() # inspect specific difference # between categories aov(Length ~ Material, data = j_data3) %>% TukeyHSD() %>% broom::tidy() %>% * arrange(adj.p.value) ``` ] .right-output-anova-4-auto[ ``` # A tibble: 2 x 6 term df sumsq meansq statistic p.value <chr> <dbl> <dbl> <dbl> <dbl> <dbl> 1 Material 10 5641. 564. 15.1 7.66e-27 2 Residuals 4815 180269. 37.4 NA NA ``` ``` # A tibble: 55 x 6 term comparison estimate conf.low conf.high adj.p.value <chr> <chr> <dbl> <dbl> <dbl> <dbl> 1 Material Volcanic-Chert 4.29 2.96 5.62 0.0000000243 2 Material Unknown-Chert 6.41 1.88 10.9 0.000278 3 Material Quartzite-Chert 4.98 1.37 8.59 0.000462 4 Material Volcanic-Silcrete 3.84 0.660 7.02 0.00487 5 Material Unknown-Silcrete 5.96 0.586 11.3 0.0158 6 Material Silcrete-Quartzite -4.53 -9.16 0.0904 0.0604 7 Material Unknown-Quartz 5.32 -1.26 11.9 0.246 8 Material Quartzite-Quartz 3.90 -2.09 9.88 0.579 9 Material Volcanic-Quartz 3.20 -1.75 8.16 0.589 10 Material FGS-Chert 11.0 -8.71 30.7 0.782 # … with 45 more rows ``` ] --- class: split-40 count: false Inspect the ANOVA output .left-code-anova-4-auto[ ```r # get the F statistic, # degrees of freedom, and p-value aov(Length ~ Material, data = j_data3) %>% broom::tidy() # inspect specific difference # between categories aov(Length ~ Material, data = j_data3) %>% TukeyHSD() %>% broom::tidy() %>% arrange(adj.p.value) %>% * select(comparison, * adj.p.value) ``` ] .right-output-anova-4-auto[ ``` # A tibble: 2 x 6 term df sumsq meansq statistic p.value <chr> <dbl> <dbl> <dbl> <dbl> <dbl> 1 Material 10 5641. 564. 15.1 7.66e-27 2 Residuals 4815 180269. 37.4 NA NA ``` ``` # A tibble: 55 x 2 comparison adj.p.value <chr> <dbl> 1 Volcanic-Chert 0.0000000243 2 Unknown-Chert 0.000278 3 Quartzite-Chert 0.000462 4 Volcanic-Silcrete 0.00487 5 Unknown-Silcrete 0.0158 6 Silcrete-Quartzite 0.0604 7 Unknown-Quartz 0.246 8 Quartzite-Quartz 0.579 9 Volcanic-Quartz 0.589 10 FGS-Chert 0.782 # … with 45 more rows ``` ] <style> .left-code-anova-4-auto { color: #777; width: 38%; height: 92%; float: left; font-size: 80% } .right-output-anova-4-auto { width: 60%; float: right; padding-left: 1%; } </style> --- class: split-40 count: false Visualise the ANOVA output .left-code-anova-5-auto[ ```r # plot *aov(Length ~ Material, * data = j_data3) ``` ] .right-output-anova-5-auto[ ``` Call: aov(formula = Length ~ Material, data = j_data3) Terms: Material Residuals Sum of Squares 5640.79 180269.35 Deg. of Freedom 10 4815 Residual standard error: 6.118751 Estimated effects may be unbalanced ``` ] --- class: split-40 count: false Visualise the ANOVA output .left-code-anova-5-auto[ ```r # plot aov(Length ~ Material, data = j_data3) %>% * TukeyHSD() ``` ] .right-output-anova-5-auto[ ``` Tukey multiple comparisons of means 95% family-wise confidence level Fit: aov(formula = Length ~ Material, data = j_data3) $Material diff lwr upr p adj Chert-Brecchert -1.9466973 -21.6525949 17.75920033 0.9999999 Cortical-Brecchert -1.1133333 -23.8651968 21.63853014 1.0000000 Dehydchert-Brecchert 0.5100000 -27.3552281 28.37522810 1.0000000 FGS-Brecchert 9.0500000 -18.8152281 36.91522810 0.9939875 Obsidian-Brecchert 0.6654545 -19.9143803 21.24528939 1.0000000 Quartz-Brecchert -0.8588235 -21.1337551 19.41610802 1.0000000 Quartzite-Brecchert 3.0370000 -16.9923947 23.06639467 0.9999934 Silcrete-Brecchert -1.4965217 -21.4132326 18.42018909 1.0000000 Unknown-Brecchert 4.4631579 -15.7524033 24.67871909 0.9997802 Volcanic-Brecchert 2.3448918 -17.4014026 22.09118615 0.9999994 Cortical-Chert 0.8333639 -10.5463880 12.21311588 1.0000000 Dehydchert-Chert 2.4566973 -17.2492003 22.16259486 0.9999990 FGS-Chert 10.9966973 -8.7092003 30.70259486 0.7822430 Obsidian-Chert 2.6121518 -3.3360466 8.56035027 0.9451831 Quartz-Chert 1.0878737 -3.7000602 5.87580773 0.9997153 Quartzite-Chert 4.9836973 1.3742494 8.59314510 0.0004617 Silcrete-Chert 0.4501755 -2.4698986 3.37024965 0.9999922 Unknown-Chert 6.4098552 1.8799129 10.93979745 0.0002781 Volcanic-Chert 4.2915890 2.9620762 5.62110188 0.0000000 Dehydchert-Cortical 1.6233333 -21.1285301 24.37519681 1.0000000 FGS-Cortical 10.1633333 -12.5885301 32.91519681 0.9387607 Obsidian-Cortical 1.7787879 -11.0549940 14.61256972 0.9999972 Quartz-Cortical 0.2545098 -12.0844206 12.59344020 1.0000000 Quartzite-Cortical 4.1503333 -7.7808445 16.08151119 0.9896880 Silcrete-Cortical -0.3831884 -12.1242158 11.35783899 1.0000000 Unknown-Cortical 5.5764912 -6.6646390 17.81762143 0.9305883 Volcanic-Cortical 3.4582251 -7.9913380 14.90778824 0.9966652 FGS-Dehydchert 8.5400000 -19.3252281 36.40522810 0.9962406 Obsidian-Dehydchert 0.1554545 -20.4243803 20.73528939 1.0000000 Quartz-Dehydchert -1.3688235 -21.6437551 18.90610802 1.0000000 Quartzite-Dehydchert 2.5270000 -17.5023947 22.55639467 0.9999989 Silcrete-Dehydchert -2.0065217 -21.9232326 17.91018909 0.9999999 Unknown-Dehydchert 3.9531579 -16.2624033 24.16871909 0.9999275 Volcanic-Dehydchert 1.8348918 -17.9114026 21.58118615 0.9999999 Obsidian-FGS -8.3845455 -28.9643803 12.19528939 0.9669934 Quartz-FGS -9.9088235 -30.1837551 10.36610802 0.8934486 Quartzite-FGS -6.0130000 -26.0423947 14.01639467 0.9968285 Silcrete-FGS -10.5465217 -30.4632326 9.37018909 0.8332796 Unknown-FGS -4.5868421 -24.8024033 15.62871909 0.9997188 Volcanic-FGS -6.7051082 -26.4514026 13.04118615 0.9914510 Quartz-Obsidian -1.5242781 -9.1486806 6.10012446 0.9999111 Quartzite-Obsidian 2.3715455 -4.5736195 9.31671038 0.9910695 Silcrete-Obsidian -2.1619763 -8.7751476 4.45119504 0.9936637 Unknown-Obsidian 3.7977033 -3.6673874 11.26279405 0.8659341 Volcanic-Obsidian 1.6794372 -4.4012541 7.76012852 0.9984250 Quartzite-Quartz 3.8958235 -2.0856949 9.87734197 0.5792526 Silcrete-Quartz -0.6376982 -6.2303078 4.95491138 0.9999996 Unknown-Quartz 5.3219814 -1.2560750 11.90003784 0.2460217 Volcanic-Quartz 3.2037153 -1.7478555 8.15528612 0.5892819 Silcrete-Quartzite -4.5335217 -9.1574901 0.09044666 0.0604008 Unknown-Quartzite 1.4261579 -4.3509199 7.20323570 0.9994046 Volcanic-Quartzite -0.6921082 -4.5159617 3.13174524 0.9999647 Unknown-Silcrete 5.9596796 0.5862871 11.33307216 0.0157827 Volcanic-Silcrete 3.8414135 0.6601285 7.02269856 0.0048717 Volcanic-Unknown -2.1182661 -6.8208312 2.58429900 0.9353882 ``` ] --- class: split-40 count: false Visualise the ANOVA output .left-code-anova-5-auto[ ```r # plot aov(Length ~ Material, data = j_data3) %>% TukeyHSD() %>% * broom::tidy() ``` ] .right-output-anova-5-auto[ ``` # A tibble: 55 x 6 term comparison estimate conf.low conf.high adj.p.value <chr> <chr> <dbl> <dbl> <dbl> <dbl> 1 Material Chert-Brecchert -1.95 -21.7 17.8 1.00 2 Material Cortical-Brecchert -1.11 -23.9 21.6 1.00 3 Material Dehydchert-Brecchert 0.51 -27.4 28.4 1 4 Material FGS-Brecchert 9.05 -18.8 36.9 0.994 5 Material Obsidian-Brecchert 0.665 -19.9 21.2 1.00 6 Material Quartz-Brecchert -0.859 -21.1 19.4 1.00 7 Material Quartzite-Brecchert 3.04 -17.0 23.1 1.00 8 Material Silcrete-Brecchert -1.50 -21.4 18.4 1.00 9 Material Unknown-Brecchert 4.46 -15.8 24.7 1.00 10 Material Volcanic-Brecchert 2.34 -17.4 22.1 1.00 # … with 45 more rows ``` ] --- class: split-40 count: false Visualise the ANOVA output .left-code-anova-5-auto[ ```r # plot aov(Length ~ Material, data = j_data3) %>% TukeyHSD() %>% broom::tidy() %>% * ggplot(aes(x = fct_reorder(comparison, * estimate), * y = estimate)) ``` ] .right-output-anova-5-auto[ <img src="stat-inference-and-exploration-for-archaeologists_files/figure-html/anova-5_auto_4_output-1.png" width="432" /> ] --- class: split-40 count: false Visualise the ANOVA output .left-code-anova-5-auto[ ```r # plot aov(Length ~ Material, data = j_data3) %>% TukeyHSD() %>% broom::tidy() %>% ggplot(aes(x = fct_reorder(comparison, estimate), y = estimate)) + * geom_pointrange(aes(ymin = conf.low, * ymax = conf.high, * color = adj.p.value < 0.05)) ``` ] .right-output-anova-5-auto[ <img src="stat-inference-and-exploration-for-archaeologists_files/figure-html/anova-5_auto_5_output-1.png" width="432" /> ] --- class: split-40 count: false Visualise the ANOVA output .left-code-anova-5-auto[ ```r # plot aov(Length ~ Material, data = j_data3) %>% TukeyHSD() %>% broom::tidy() %>% ggplot(aes(x = fct_reorder(comparison, estimate), y = estimate)) + geom_pointrange(aes(ymin = conf.low, ymax = conf.high, color = adj.p.value < 0.05)) + * geom_hline(yintercept = 0, * linetype = "dashed") ``` ] .right-output-anova-5-auto[ <img src="stat-inference-and-exploration-for-archaeologists_files/figure-html/anova-5_auto_6_output-1.png" width="432" /> ] --- class: split-40 count: false Visualise the ANOVA output .left-code-anova-5-auto[ ```r # plot aov(Length ~ Material, data = j_data3) %>% TukeyHSD() %>% broom::tidy() %>% ggplot(aes(x = fct_reorder(comparison, estimate), y = estimate)) + geom_pointrange(aes(ymin = conf.low, ymax = conf.high, color = adj.p.value < 0.05)) + geom_hline(yintercept = 0, linetype = "dashed") + * coord_flip() ``` ] .right-output-anova-5-auto[ <img src="stat-inference-and-exploration-for-archaeologists_files/figure-html/anova-5_auto_7_output-1.png" width="432" /> ] --- class: split-40 count: false Visualise the ANOVA output .left-code-anova-5-auto[ ```r # plot aov(Length ~ Material, data = j_data3) %>% TukeyHSD() %>% broom::tidy() %>% ggplot(aes(x = fct_reorder(comparison, estimate), y = estimate)) + geom_pointrange(aes(ymin = conf.low, ymax = conf.high, color = adj.p.value < 0.05)) + geom_hline(yintercept = 0, linetype = "dashed") + coord_flip() + * labs(x = NULL, * colour = "padj < 0.05") ``` ] .right-output-anova-5-auto[ <img src="stat-inference-and-exploration-for-archaeologists_files/figure-html/anova-5_auto_8_output-1.png" width="432" /> ] --- class: split-40 count: false Visualise the ANOVA output .left-code-anova-5-auto[ ```r # plot aov(Length ~ Material, data = j_data3) %>% TukeyHSD() %>% broom::tidy() %>% ggplot(aes(x = fct_reorder(comparison, estimate), y = estimate)) + geom_pointrange(aes(ymin = conf.low, ymax = conf.high, color = adj.p.value < 0.05)) + geom_hline(yintercept = 0, linetype = "dashed") + coord_flip() + labs(x = NULL, colour = "padj < 0.05") + * theme_bw(base_size = 10) ``` ] .right-output-anova-5-auto[ <img src="stat-inference-and-exploration-for-archaeologists_files/figure-html/anova-5_auto_9_output-1.png" width="432" /> ] <style> .left-code-anova-5-auto { color: #777; width: 60%; height: 92%; float: left; font-size: 80% } .right-output-anova-5-auto { width: 38%; float: right; padding-left: 1%; } </style> --- class: left, top background-image: url(https://images.unsplash.com/photo-1505778276668-26b3ff7af103?ixlib=rb-1.2.1&ixid=eyJhcHBfaWQiOjEyMDd9&auto=format&fit=crop&w=1643&q=80) background-size: cover # Exploring the data with PCA and k-means --- # Let's do a Principal Components Analysis to reduce our multivariate data into smaller set of variables to observe trends, clusters and outliers -- Our goal is to find some linear combinations of original variables that captures the maximum variance in the data set. These determine the which combinations of variables contain the highest variability in our data. --- class: left, bottom background-image: url(figures/pca-1.png) background-size: contain --- class: left, bottom background-image: url(figures/pca-2.gif) background-size: contain --- class: left, bottom background-image: url(figures/pca-3.gif) background-size: contain --- class: left, bottom background-image: url(figures/pca-3.gif) background-size: contain --- class: left, bottom background-image: url(figures/pca-4.gif) background-size: contain --- class: left, bottom background-image: url(figures/pca-5.png) background-size: contain --- class: left, bottom background-image: url(figures/pca-animated-1.gif) background-size: contain --- class: center, left .pull-left[ 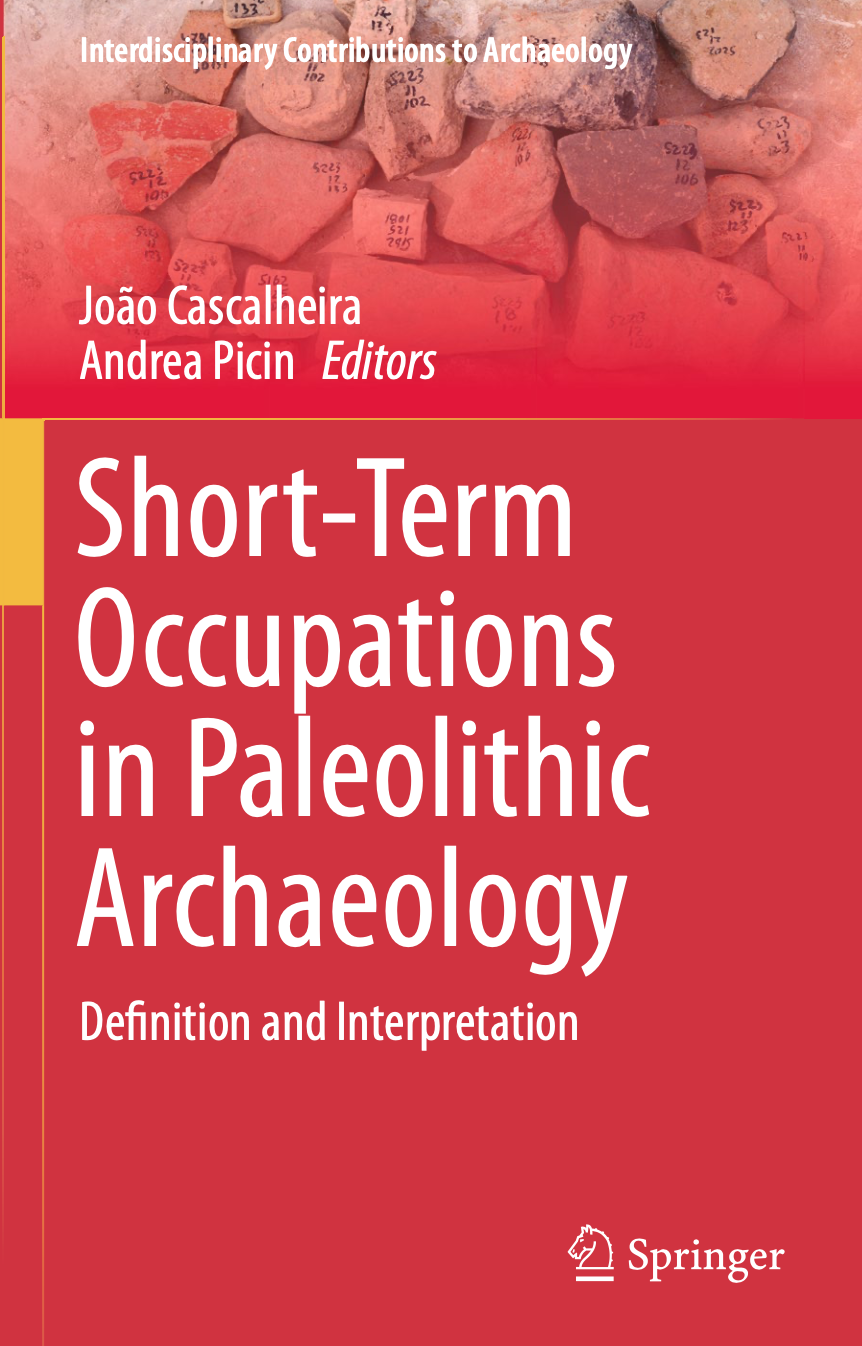 ] .pull-right[ 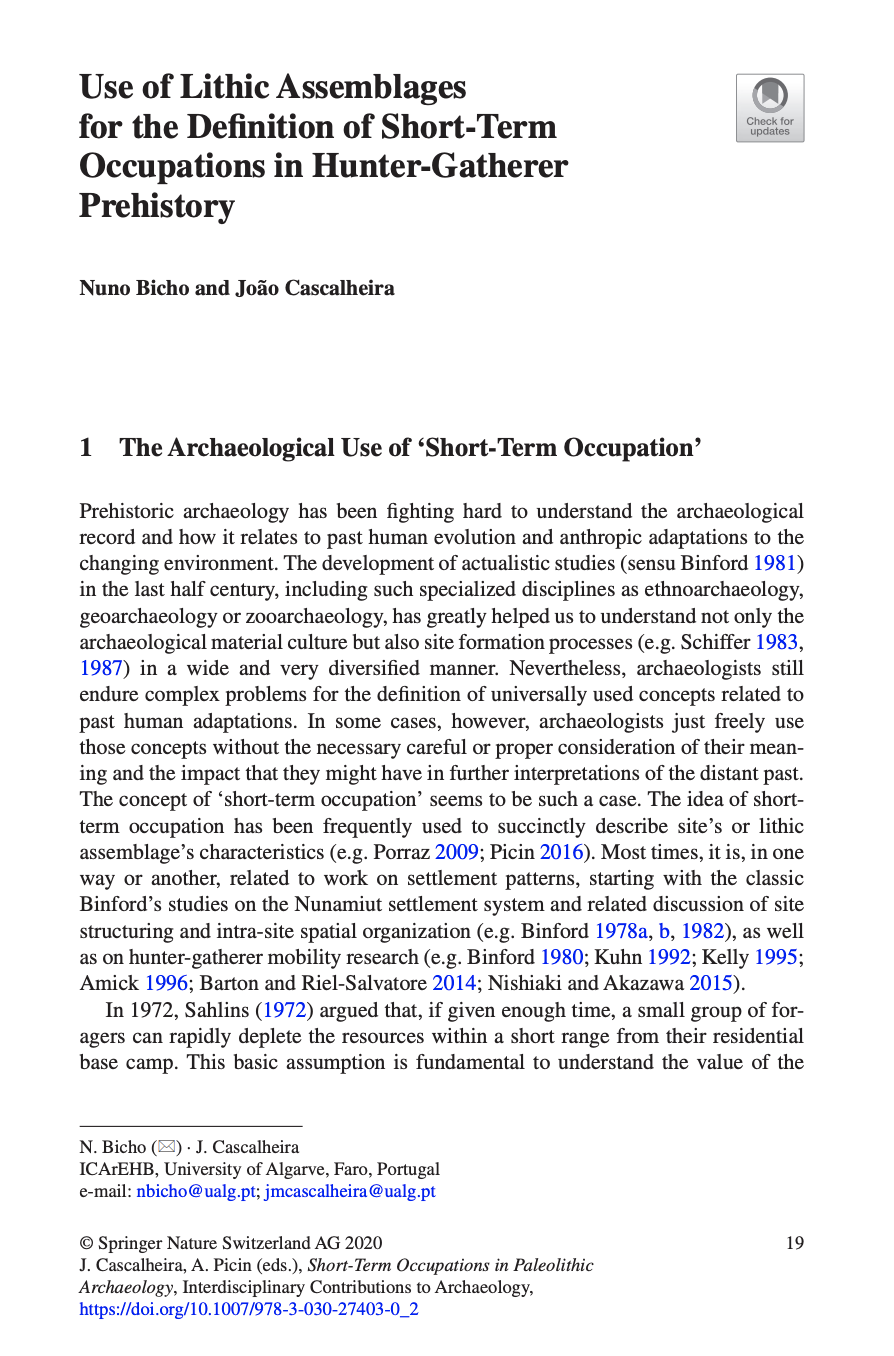 ] --- # Can we use stone artefact assemblage attributes to identify short-term occupations at Palaeolithic sites? --- class: split-40 count: false Get and prepare data for PCA .left-code-pca-1-auto[ ```r *library(rio) ``` ] .right-output-pca-1-auto[ ] --- class: split-40 count: false Get and prepare data for PCA .left-code-pca-1-auto[ ```r library(rio) # get the prepped data *nb_data <- import("https://bit.ly/2XFcOB5", * setclass = "tibble") ``` ] .right-output-pca-1-auto[ ] --- class: split-40 count: false Get and prepare data for PCA .left-code-pca-1-auto[ ```r library(rio) # get the prepped data nb_data <- import("https://bit.ly/2XFcOB5", setclass = "tibble") # inspect the data *str(nb_data) ``` ] .right-output-pca-1-auto[ ``` tibble [16 × 9] (S3: tbl_df/tbl/data.frame) $ chipFreq : num [1:16] 0.415 0.356 0.264 0.387 0.44 ... $ coreFreq : num [1:16] 0.041 0.0161 0.0461 0.0142 0.0181 ... $ blankFreq : num [1:16] 0.435 0.543 0.34 0.493 0.463 ... $ retFreq : num [1:16] 0.0996 0.0605 0.3149 0.0781 0.0531 ... $ featFreq : num [1:16] 0 2.22 0 0 0 ... $ toolDiv : num [1:16] 2.66 3.69 1.87 3.14 3.38 ... $ lithDens : num [1:16] 4096 3304 3359 2393 1291 ... $ EstimatedArea: int [1:16] 50 8 70 70 40 70 60 100 50 100 ... $ Sites : chr [1:16] "AR I" "CPM III Trench" "CPM I Upper" "CPM II Upper" ... ``` ] --- class: split-40 count: false Get and prepare data for PCA .left-code-pca-1-auto[ ```r library(rio) # get the prepped data nb_data <- import("https://bit.ly/2XFcOB5", setclass = "tibble") # inspect the data str(nb_data) # prepare the data *nb_data_rownames <- nb_data ``` ] .right-output-pca-1-auto[ ``` tibble [16 × 9] (S3: tbl_df/tbl/data.frame) $ chipFreq : num [1:16] 0.415 0.356 0.264 0.387 0.44 ... $ coreFreq : num [1:16] 0.041 0.0161 0.0461 0.0142 0.0181 ... $ blankFreq : num [1:16] 0.435 0.543 0.34 0.493 0.463 ... $ retFreq : num [1:16] 0.0996 0.0605 0.3149 0.0781 0.0531 ... $ featFreq : num [1:16] 0 2.22 0 0 0 ... $ toolDiv : num [1:16] 2.66 3.69 1.87 3.14 3.38 ... $ lithDens : num [1:16] 4096 3304 3359 2393 1291 ... $ EstimatedArea: int [1:16] 50 8 70 70 40 70 60 100 50 100 ... $ Sites : chr [1:16] "AR I" "CPM III Trench" "CPM I Upper" "CPM II Upper" ... ``` ] --- class: split-40 count: false Get and prepare data for PCA .left-code-pca-1-auto[ ```r library(rio) # get the prepped data nb_data <- import("https://bit.ly/2XFcOB5", setclass = "tibble") # inspect the data str(nb_data) # prepare the data nb_data_rownames <- nb_data %>% * column_to_rownames(var="Sites") ``` ] .right-output-pca-1-auto[ ``` tibble [16 × 9] (S3: tbl_df/tbl/data.frame) $ chipFreq : num [1:16] 0.415 0.356 0.264 0.387 0.44 ... $ coreFreq : num [1:16] 0.041 0.0161 0.0461 0.0142 0.0181 ... $ blankFreq : num [1:16] 0.435 0.543 0.34 0.493 0.463 ... $ retFreq : num [1:16] 0.0996 0.0605 0.3149 0.0781 0.0531 ... $ featFreq : num [1:16] 0 2.22 0 0 0 ... $ toolDiv : num [1:16] 2.66 3.69 1.87 3.14 3.38 ... $ lithDens : num [1:16] 4096 3304 3359 2393 1291 ... $ EstimatedArea: int [1:16] 50 8 70 70 40 70 60 100 50 100 ... $ Sites : chr [1:16] "AR I" "CPM III Trench" "CPM I Upper" "CPM II Upper" ... ``` ] <style> .left-code-pca-1-auto { color: #777; width: 60%; height: 92%; float: left; font-size: 80% } .right-output-pca-1-auto { width: 38%; float: right; padding-left: 1%; } </style> --- class: split-40 count: false Compute PCA .left-code-pca-2-auto[ ```r *library(FactoMineR) ``` ] .right-output-pca-2-auto[ ] --- class: split-40 count: false Compute PCA .left-code-pca-2-auto[ ```r library(FactoMineR) # compute PCA *res.pca <- PCA(nb_data_rownames, * graph = FALSE) ``` ] .right-output-pca-2-auto[ ] --- class: split-40 count: false Compute PCA .left-code-pca-2-auto[ ```r library(FactoMineR) # compute PCA res.pca <- PCA(nb_data_rownames, graph = FALSE) # inspect eigenvalues *res.pca$eig ``` ] .right-output-pca-2-auto[ ``` eigenvalue percentage of variance cumulative percentage of variance comp 1 2.76443434 34.555429 34.55543 comp 2 1.94019355 24.252419 58.80785 comp 3 1.24621394 15.577674 74.38552 comp 4 1.07640752 13.455094 87.84062 comp 5 0.60652553 7.581569 95.42219 comp 6 0.25615026 3.201878 98.62406 comp 7 0.08412005 1.051501 99.67556 comp 8 0.02595480 0.324435 100.00000 ``` ] --- class: split-40 count: false Compute PCA .left-code-pca-2-auto[ ```r library(FactoMineR) # compute PCA res.pca <- PCA(nb_data_rownames, graph = FALSE) # inspect eigenvalues res.pca$eig ``` ] .right-output-pca-2-auto[ ``` eigenvalue percentage of variance cumulative percentage of variance comp 1 2.76443434 34.555429 34.55543 comp 2 1.94019355 24.252419 58.80785 comp 3 1.24621394 15.577674 74.38552 comp 4 1.07640752 13.455094 87.84062 comp 5 0.60652553 7.581569 95.42219 comp 6 0.25615026 3.201878 98.62406 comp 7 0.08412005 1.051501 99.67556 comp 8 0.02595480 0.324435 100.00000 ``` ] <style> .left-code-pca-2-auto { color: #777; width: 38%; height: 92%; float: left; font-size: 80% } .right-output-pca-2-auto { width: 60%; float: right; padding-left: 1%; } </style> --- class: split-40 count: false Inspect PCA .left-code-pca-3-auto[ ```r # inspect distribution of PCs *library(factoextra) ``` ] .right-output-pca-3-auto[ ] --- class: split-40 count: false Inspect PCA .left-code-pca-3-auto[ ```r # inspect distribution of PCs library(factoextra) *fviz_screeplot(res.pca) ``` ] .right-output-pca-3-auto[ <img src="stat-inference-and-exploration-for-archaeologists_files/figure-html/pca-3_auto_2_output-1.png" width="432" /> ] --- class: split-40 count: false Inspect PCA .left-code-pca-3-auto[ ```r # inspect distribution of PCs library(factoextra) fviz_screeplot(res.pca) ``` ] .right-output-pca-3-auto[ <img src="stat-inference-and-exploration-for-archaeologists_files/figure-html/pca-3_auto_3_output-1.png" width="432" /> ] <style> .left-code-pca-3-auto { color: #777; width: 38%; height: 92%; float: left; font-size: 80% } .right-output-pca-3-auto { width: 60%; float: right; padding-left: 1%; } </style> --- class: split-40 count: false Contributions of variables to the PCs .left-code-pca-3b-auto[ ```r # Contributions of # variables to PC1 *fviz_contrib(res.pca, * choice = "var", * axes = 1, * top = 10) ``` ] .right-output-pca-3b-auto[ <img src="stat-inference-and-exploration-for-archaeologists_files/figure-html/pca-3b_auto_1_output-1.png" width="432" /> ] --- class: split-40 count: false Contributions of variables to the PCs .left-code-pca-3b-auto[ ```r # Contributions of # variables to PC1 fviz_contrib(res.pca, choice = "var", axes = 1, top = 10) ``` ] .right-output-pca-3b-auto[ <img src="stat-inference-and-exploration-for-archaeologists_files/figure-html/pca-3b_auto_2_output-1.png" width="432" /> ] <style> .left-code-pca-3b-auto { color: #777; width: 38%; height: 92%; float: left; font-size: 80% } .right-output-pca-3b-auto { width: 60%; float: right; padding-left: 1%; } </style> --- class: split-40 count: false Visualise PCA results .left-code-pca-4-auto[ ```r # draw biplot *fviz_pca_biplot(res.pca) ``` ] .right-output-pca-4-auto[ <img src="stat-inference-and-exploration-for-archaeologists_files/figure-html/pca-4_auto_1_output-1.png" width="432" /> ] --- class: split-40 count: false Visualise PCA results .left-code-pca-4-auto[ ```r # draw biplot fviz_pca_biplot(res.pca) ``` ] .right-output-pca-4-auto[ <img src="stat-inference-and-exploration-for-archaeologists_files/figure-html/pca-4_auto_2_output-1.png" width="432" /> ] <style> .left-code-pca-4-auto { color: #777; width: 45%; height: 92%; float: left; font-size: 80% } .right-output-pca-4-auto { width: 50%; float: right; padding-left: 1%; } </style> --- # Your turn: PCA ```r # get the prepped data nb_data <- rio::import("https://bit.ly/2XFcOB5", setclass = "tibble") # prepare the data nb_data_rownames <- nb_data %>% column_to_rownames(var="Sites") ``` ```r library(FactoMineR) # compute PCA res.pca <- PCA(nb_data_rownames, graph = FALSE) # inspect eigenvalues res.pca$eig ``` ```r # draw biplot fviz_pca_biplot(res.pca) ```
07
:
00
--- class: left, bottom background-image: url(figures/allison-horst-kmeans-1.jpg) background-size: contain --- class: left, bottom background-image: url(figures/allison-horst-kmeans-2.jpg) background-size: contain --- class: left, bottom background-image: url(figures/allison-horst-kmeans-3.jpg) background-size: contain --- class: left, bottom background-image: url(figures/allison-horst-kmeans-4.jpg) background-size: contain --- class: left, bottom background-image: url(figures/allison-horst-kmeans-5.jpg) background-size: contain --- class: left, bottom background-image: url(figures/allison-horst-kmeans-6.jpg) background-size: contain --- class: left, bottom background-image: url(figures/allison-horst-kmeans-7.jpg) background-size: contain --- class: left, bottom background-image: url(figures/allison-horst-kmeans-8.jpg) background-size: contain --- class: left, bottom background-image: url(figures/allison-horst-kmeans-9.jpg) background-size: contain --- class: left, bottom background-image: url(figures/allison-horst-kmeans-10.jpg) background-size: contain --- class: left, bottom background-image: url(figures/allison-horst-kmeans-11.jpg) background-size: contain --- class: left, bottom background-image: url(figures/allison-horst-kmeans-12.jpg) background-size: contain --- class: left, bottom background-image: url(figures/kmeans-animation.gif) background-size: contain --- # Let's do a k-means analysis to see what groups exist in our data. --- class: split-40 count: false Prepare and inspect our data .left-code-k-1-auto[ ```r # filter the data to # focus on our question # prepare data *j_data4 <- j_data ``` ] .right-output-k-1-auto[ ] --- class: split-40 count: false Prepare and inspect our data .left-code-k-1-auto[ ```r # filter the data to # focus on our question # prepare data j_data4 <- j_data %>% * filter(Material %in% c("Silcrete")) ``` ] .right-output-k-1-auto[ ] --- class: split-40 count: false Prepare and inspect our data .left-code-k-1-auto[ ```r # filter the data to # focus on our question # prepare data j_data4 <- j_data %>% filter(Material %in% c("Silcrete")) %>% * select(Length, * Thick, * Width) ``` ] .right-output-k-1-auto[ ] --- class: split-40 count: false Prepare and inspect our data .left-code-k-1-auto[ ```r # filter the data to # focus on our question # prepare data j_data4 <- j_data %>% filter(Material %in% c("Silcrete")) %>% select(Length, Thick, Width) # inspect *ggplot(j_data4) ``` ] .right-output-k-1-auto[ <img src="stat-inference-and-exploration-for-archaeologists_files/figure-html/k-1_auto_4_output-1.png" width="432" /> ] --- class: split-40 count: false Prepare and inspect our data .left-code-k-1-auto[ ```r # filter the data to # focus on our question # prepare data j_data4 <- j_data %>% filter(Material %in% c("Silcrete")) %>% select(Length, Thick, Width) # inspect ggplot(j_data4) + * aes(Length, * Thick, * colour = Width) ``` ] .right-output-k-1-auto[ <img src="stat-inference-and-exploration-for-archaeologists_files/figure-html/k-1_auto_5_output-1.png" width="432" /> ] --- class: split-40 count: false Prepare and inspect our data .left-code-k-1-auto[ ```r # filter the data to # focus on our question # prepare data j_data4 <- j_data %>% filter(Material %in% c("Silcrete")) %>% select(Length, Thick, Width) # inspect ggplot(j_data4) + aes(Length, Thick, colour = Width) + * geom_point() ``` ] .right-output-k-1-auto[ <img src="stat-inference-and-exploration-for-archaeologists_files/figure-html/k-1_auto_6_output-1.png" width="432" /> ] --- class: split-40 count: false Prepare and inspect our data .left-code-k-1-auto[ ```r # filter the data to # focus on our question # prepare data j_data4 <- j_data %>% filter(Material %in% c("Silcrete")) %>% select(Length, Thick, Width) # inspect ggplot(j_data4) + aes(Length, Thick, colour = Width) + geom_point() + * theme_minimal() ``` ] .right-output-k-1-auto[ <img src="stat-inference-and-exploration-for-archaeologists_files/figure-html/k-1_auto_7_output-1.png" width="432" /> ] <style> .left-code-k-1-auto { color: #777; width: 60%; height: 92%; float: left; font-size: 80% } .right-output-k-1-auto { width: 38%; float: right; padding-left: 1%; } </style> --- class: split-40 count: false Find our how many clusters we need .left-code-k-2-auto[ ```r # find an appropriate # number of clusters to use. *library(factoextra) ``` ] .right-output-k-2-auto[ ] --- class: split-40 count: false Find our how many clusters we need .left-code-k-2-auto[ ```r # find an appropriate # number of clusters to use. library(factoextra) *fviz_nbclust(j_data4, * kmeans, * method = "wss") ``` ] .right-output-k-2-auto[ <img src="stat-inference-and-exploration-for-archaeologists_files/figure-html/k-2_auto_2_output-1.png" width="432" /> ] --- class: split-40 count: false Find our how many clusters we need .left-code-k-2-auto[ ```r # find an appropriate # number of clusters to use. library(factoextra) fviz_nbclust(j_data4, kmeans, method = "wss") + * geom_vline(xintercept = 4) ``` ] .right-output-k-2-auto[ <img src="stat-inference-and-exploration-for-archaeologists_files/figure-html/k-2_auto_3_output-1.png" width="432" /> ] <style> .left-code-k-2-auto { color: #777; width: 38%; height: 92%; float: left; font-size: 80% } .right-output-k-2-auto { width: 60%; float: right; padding-left: 1%; } </style> --- class: split-40 count: false Compute the cluster analysis .left-code-k-3-auto[ ```r # compute clusters *kmeans(j_data4, * centers = 4, * nstart = 50) ``` ] .right-output-k-3-auto[ ``` K-means clustering with 4 clusters of sizes 40, 14, 6, 34 Cluster means: Length Thick Width 1 11.322250 2.889250 9.880750 2 13.805714 3.504286 16.695714 3 25.871667 6.713333 18.836667 4 6.889118 2.038529 6.357941 Clustering vector: [1] 1 4 2 4 1 4 4 4 4 1 1 2 1 1 1 2 1 3 1 4 1 1 1 4 2 4 1 4 4 4 1 1 3 2 4 4 4 4 4 1 4 4 1 4 1 3 1 2 1 4 4 [52] 1 1 1 4 1 1 2 4 2 3 1 1 4 2 1 3 2 2 2 1 4 4 1 1 1 2 4 1 2 1 1 4 4 1 3 4 4 4 4 1 1 1 1 Within cluster sum of squares by cluster: [1] 559.1925 242.5442 439.3825 283.3241 (between_SS / total_SS = 70.7 %) Available components: [1] "cluster" "centers" "totss" "withinss" "tot.withinss" "betweenss" [7] "size" "iter" "ifault" ``` ] --- class: split-40 count: false Compute the cluster analysis .left-code-k-3-auto[ ```r # compute clusters kmeans(j_data4, centers = 4, nstart = 50) # view tidy output *tidy(kmeans_output) ``` ] .right-output-k-3-auto[ ``` K-means clustering with 4 clusters of sizes 14, 40, 6, 34 Cluster means: Length Thick Width 1 13.805714 3.504286 16.695714 2 11.322250 2.889250 9.880750 3 25.871667 6.713333 18.836667 4 6.889118 2.038529 6.357941 Clustering vector: [1] 2 4 1 4 2 4 4 4 4 2 2 1 2 2 2 1 2 3 2 4 2 2 2 4 1 4 2 4 4 4 2 2 3 1 4 4 4 4 4 2 4 4 2 4 2 3 2 1 2 4 4 [52] 2 2 2 4 2 2 1 4 1 3 2 2 4 1 2 3 1 1 1 2 4 4 2 2 2 1 4 2 1 2 2 4 4 2 3 4 4 4 4 2 2 2 2 Within cluster sum of squares by cluster: [1] 242.5442 559.1925 439.3825 283.3241 (between_SS / total_SS = 70.7 %) Available components: [1] "cluster" "centers" "totss" "withinss" "tot.withinss" "betweenss" [7] "size" "iter" "ifault" ``` ``` # A tibble: 4 x 6 x1 x2 x3 size withinss cluster <dbl> <dbl> <dbl> <int> <dbl> <fct> 1 13.8 3.50 16.7 14 243. 1 2 11.3 2.89 9.88 40 559. 2 3 6.89 2.04 6.36 34 283. 3 4 25.9 6.71 18.8 6 439. 4 ``` ] --- class: split-40 count: false Compute the cluster analysis .left-code-k-3-auto[ ```r # compute clusters kmeans(j_data4, centers = 4, nstart = 50) # view tidy output tidy(kmeans_output) # see which cluster each # artefact is in *augment(kmeans_output, * j_data4) ``` ] .right-output-k-3-auto[ ``` K-means clustering with 4 clusters of sizes 6, 34, 40, 14 Cluster means: Length Thick Width 1 25.871667 6.713333 18.836667 2 6.889118 2.038529 6.357941 3 11.322250 2.889250 9.880750 4 13.805714 3.504286 16.695714 Clustering vector: [1] 3 2 4 2 3 2 2 2 2 3 3 4 3 3 3 4 3 1 3 2 3 3 3 2 4 2 3 2 2 2 3 3 1 4 2 2 2 2 2 3 2 2 3 2 3 1 3 4 3 2 2 [52] 3 3 3 2 3 3 4 2 4 1 3 3 2 4 3 1 4 4 4 3 2 2 3 3 3 4 2 3 4 3 3 2 2 3 1 2 2 2 2 3 3 3 3 Within cluster sum of squares by cluster: [1] 439.3825 283.3241 559.1925 242.5442 (between_SS / total_SS = 70.7 %) Available components: [1] "cluster" "centers" "totss" "withinss" "tot.withinss" "betweenss" [7] "size" "iter" "ifault" ``` ``` # A tibble: 4 x 6 x1 x2 x3 size withinss cluster <dbl> <dbl> <dbl> <int> <dbl> <fct> 1 13.8 3.50 16.7 14 243. 1 2 11.3 2.89 9.88 40 559. 2 3 6.89 2.04 6.36 34 283. 3 4 25.9 6.71 18.8 6 439. 4 ``` ``` # A tibble: 94 x 4 Length Thick Width .cluster <dbl> <dbl> <dbl> <fct> 1 10.9 3.14 13.0 2 2 6.72 2.06 6.49 3 3 10.9 1.96 16.1 1 4 6.55 2.9 9.46 3 5 12.3 2.22 9.75 2 6 5.07 1.27 10.4 3 7 7.97 2.34 8.14 3 8 7.58 2.81 6.23 3 9 8.15 2.31 4.81 3 10 8.89 2.02 9.03 2 # … with 84 more rows ``` ] <style> .left-code-k-3-auto { color: #777; width: 38%; height: 92%; float: left; font-size: 80% } .right-output-k-3-auto { width: 60%; float: right; padding-left: 1%; } </style> --- class: split-40 count: false Visualise the clusters with the data .left-code-k-4-auto[ ```r # visualise the clusters # in our data *j_data4 ``` ] .right-output-k-4-auto[ ``` # A tibble: 94 x 3 Length Thick Width <dbl> <dbl> <dbl> 1 10.9 3.14 13.0 2 6.72 2.06 6.49 3 10.9 1.96 16.1 4 6.55 2.9 9.46 5 12.3 2.22 9.75 6 5.07 1.27 10.4 7 7.97 2.34 8.14 8 7.58 2.81 6.23 9 8.15 2.31 4.81 10 8.89 2.02 9.03 # … with 84 more rows ``` ] --- class: split-40 count: false Visualise the clusters with the data .left-code-k-4-auto[ ```r # visualise the clusters # in our data j_data4 %>% * mutate(cluster = kmeans_output$cluster) ``` ] .right-output-k-4-auto[ ``` # A tibble: 94 x 4 Length Thick Width cluster <dbl> <dbl> <dbl> <int> 1 10.9 3.14 13.0 2 2 6.72 2.06 6.49 3 3 10.9 1.96 16.1 1 4 6.55 2.9 9.46 3 5 12.3 2.22 9.75 2 6 5.07 1.27 10.4 3 7 7.97 2.34 8.14 3 8 7.58 2.81 6.23 3 9 8.15 2.31 4.81 3 10 8.89 2.02 9.03 2 # … with 84 more rows ``` ] --- class: split-40 count: false Visualise the clusters with the data .left-code-k-4-auto[ ```r # visualise the clusters # in our data j_data4 %>% mutate(cluster = kmeans_output$cluster) %>% * ggplot(aes(Length, * Thick, * color = factor(cluster), * label = cluster)) ``` ] .right-output-k-4-auto[ <img src="stat-inference-and-exploration-for-archaeologists_files/figure-html/k-4_auto_3_output-1.png" width="432" /> ] --- class: split-40 count: false Visualise the clusters with the data .left-code-k-4-auto[ ```r # visualise the clusters # in our data j_data4 %>% mutate(cluster = kmeans_output$cluster) %>% ggplot(aes(Length, Thick, color = factor(cluster), label = cluster)) + * geom_text() ``` ] .right-output-k-4-auto[ <img src="stat-inference-and-exploration-for-archaeologists_files/figure-html/k-4_auto_4_output-1.png" width="432" /> ] --- class: split-40 count: false Visualise the clusters with the data .left-code-k-4-auto[ ```r # visualise the clusters # in our data j_data4 %>% mutate(cluster = kmeans_output$cluster) %>% ggplot(aes(Length, Thick, color = factor(cluster), label = cluster)) + geom_text() + * theme_minimal() ``` ] .right-output-k-4-auto[ <img src="stat-inference-and-exploration-for-archaeologists_files/figure-html/k-4_auto_5_output-1.png" width="432" /> ] <style> .left-code-k-4-auto { color: #777; width: 65%; height: 92%; float: left; font-size: 80% } .right-output-k-4-auto { width: 30%; float: right; padding-left: 1%; } </style> --- class: split-40 count: false Visualise the clusters with a PCA .left-code-k-5-auto[ ```r # visualise the clusters in PCA *fviz_cluster(kmeans_output, * data = j_data4) ``` ] .right-output-k-5-auto[ <img src="stat-inference-and-exploration-for-archaeologists_files/figure-html/k-5_auto_1_output-1.png" width="432" /> ] --- class: split-40 count: false Visualise the clusters with a PCA .left-code-k-5-auto[ ```r # visualise the clusters in PCA fviz_cluster(kmeans_output, data = j_data4) + * theme_minimal() ``` ] .right-output-k-5-auto[ <img src="stat-inference-and-exploration-for-archaeologists_files/figure-html/k-5_auto_2_output-1.png" width="432" /> ] --- class: split-40 count: false Visualise the clusters with a PCA .left-code-k-5-auto[ ```r # visualise the clusters in PCA fviz_cluster(kmeans_output, data = j_data4) + theme_minimal() ``` ] .right-output-k-5-auto[ <img src="stat-inference-and-exploration-for-archaeologists_files/figure-html/k-5_auto_3_output-1.png" width="432" /> ] <style> .left-code-k-5-auto { color: #777; width: 65%; height: 92%; float: left; font-size: 80% } .right-output-k-5-auto { width: 30%; float: right; padding-left: 1%; } </style> --- background-image: url(https://images.unsplash.com/photo-1543175451-3d57d601828c?ixlib=rb-1.2.1&ixid=eyJhcHBfaWQiOjEyMDd9&auto=format&fit=crop&w=934&q=80) background-size: cover --- # Your turn: k-means ```r j_data4 <- j_data %>% filter(Material %in% c("Silcrete")) %>% select(Length, Thick, Width) ``` ```r # find an appropriate number of clusters to use. library(factoextra) fviz_nbclust(j_data4, kmeans, method = "wss") + geom_vline(xintercept = 4) ``` ```r # compute clusters set.seed(7) kmeans_output <- kmeans(j_data4, centers = 4, nstart = 50) ``` ```r # visualise the clusters in PCA fviz_cluster(kmeans_output, data = j_data4) + theme_minimal() ```
07
:
00
--- # What have we done today? - Hypothesis testing: chi-square, t-test, ANOVA - Exploration: Principal Components Analysis - Exploration: k-means clustering --- background-image: url(https://images.unsplash.com/photo-1503980599186-9cc36eda351a?ixlib=rb-1.2.1&ixid=eyJhcHBfaWQiOjEyMDd9&auto=format&fit=crop&w=1050&q=80) background-size: cover --- background-image: url(figures/end.gif) background-size: cover --- class: inverse, middle, center The flipbooked portion of this presentation was created with the new {flipbookr} package. Get it with `remotes::install_github("EvaMaeRey/flipbookr")` ---